Last week, we discussed AI's incredible evolution in terms of its performance against humans. Almost across the board, AI has surpassed humans in a range of performance-based tasks, necessitating the development of new, more challenging benchmarks. Arguably, that degree of development could be classed as a 'good.' This follow-up article discusses the not-so-good that has resulted from AI's rapid evolution.
The recently released 2024 AI Index report by Stanford University’s Institute for Human-Centered Artificial Intelligence (HAI) comprehensively examines AI's global impact. The seventh edition of the annual report has more content than previous editions, reflecting AI's rapid evolution and growing significance in our everyday lives.
Written by an interdisciplinary team of academic and industrial experts, the 500-page report provides an independent, unbiased look at the health of AI. We've already spoken about the 'good' – now it's time to tackle the bad and the ugly.
With AI now integrated into many facets of our lives, it must be responsible for its contribution, especially to important sectors like education, healthcare, and finance. Yes, the addition of AI can provide advantages – optimizing processes and productivity, discovering new drugs, for example – but it also carries risks.
In short, it needs to 'get it right.' And, of course, a good deal of that responsibility falls to developers.
What is responsible AI and how's it measured?
According to the new AI Index report, truly responsible AI models must meet public expectations in key areas: data privacy, data governance, security and safety, fairness, and transparency and explainability.
Data privacy safeguards an individual's confidentiality, anonymity, and personal data. It includes the right to consent to and be informed about data usage. Data governance includes policies and procedures that ensure data quality, with a focus on ethical use. Security and safety include measures that ensure a system's reliability and minimize the risk of data misuse, cyber threats, and inherent system errors.
Fairness means using algorithms that avoid bias and discrimination and align with broader societal concepts of equity. Transparency is openly sharing data sources and algorithmic decisions, as well as considering how AI systems are monitored and managed from creation to operation. Explainability means the ability of developers to explain the rationale for their AI-related choices in understandable language.
For this year's report, Stanford researchers collaborated with Accenture to survey respondents from more than 1,000 worldwide organizations and asked them which risks they considered relevant. The result was the Global State of Responsible AI survey.
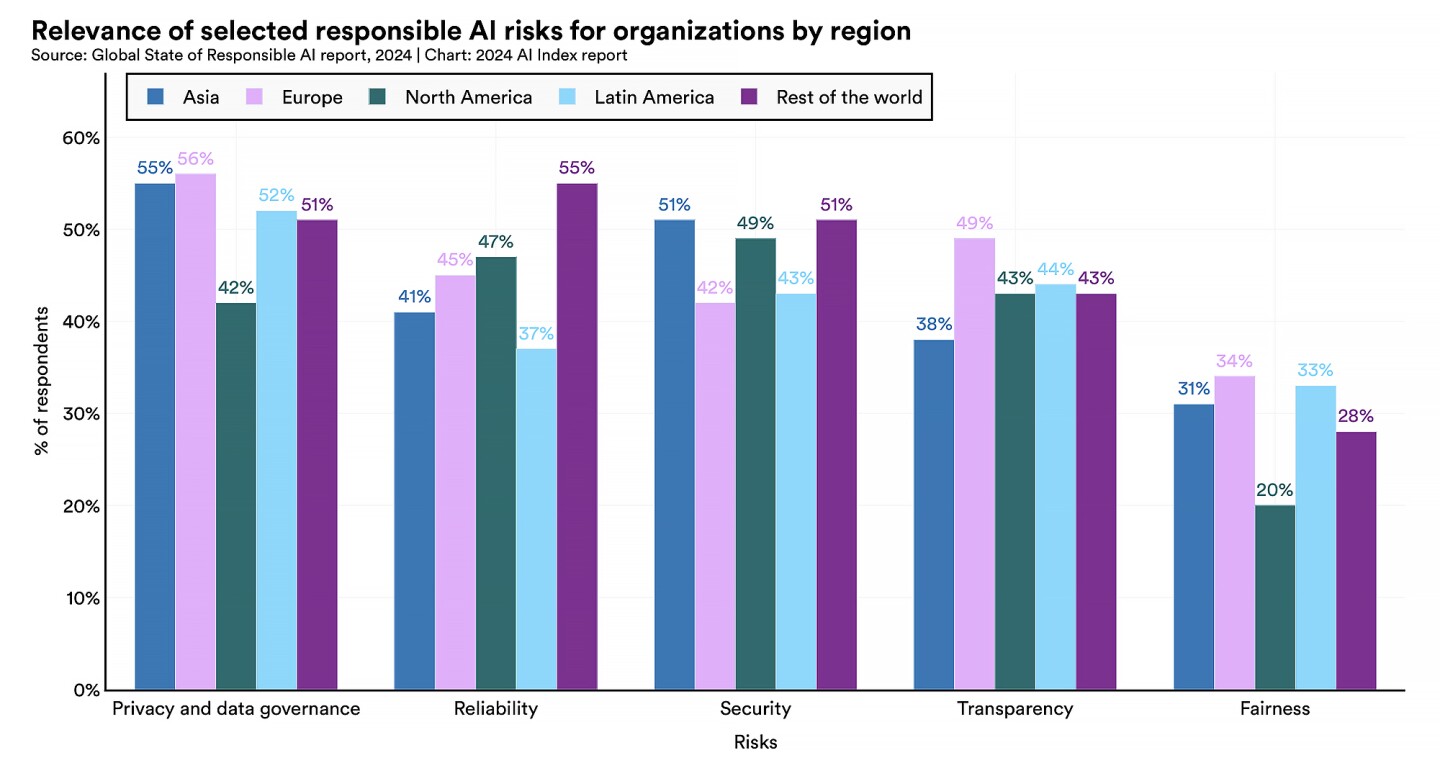
As the above chart shows, data privacy and governance risks were the highest global concern. However, more Asian (55%) and European (56%) respondents were concerned about these risks than those from North America (42%).
And while, globally, organizations were least concerned with risks to fairness, there was a stark difference between North American respondents (20%) and those from Asia (31%) and Europe (34%).
Few organizations had already implemented measures to mitigate the risks associated with the key aspects of responsible AI: 18% of companies in Europe, 17% in North America, and 25% of Asian companies.
Which AI model is the most trustworthy?
Responsibility incorporates trustworthiness. So, which large language model (LLM) did the AI Index report find most trustworthy?
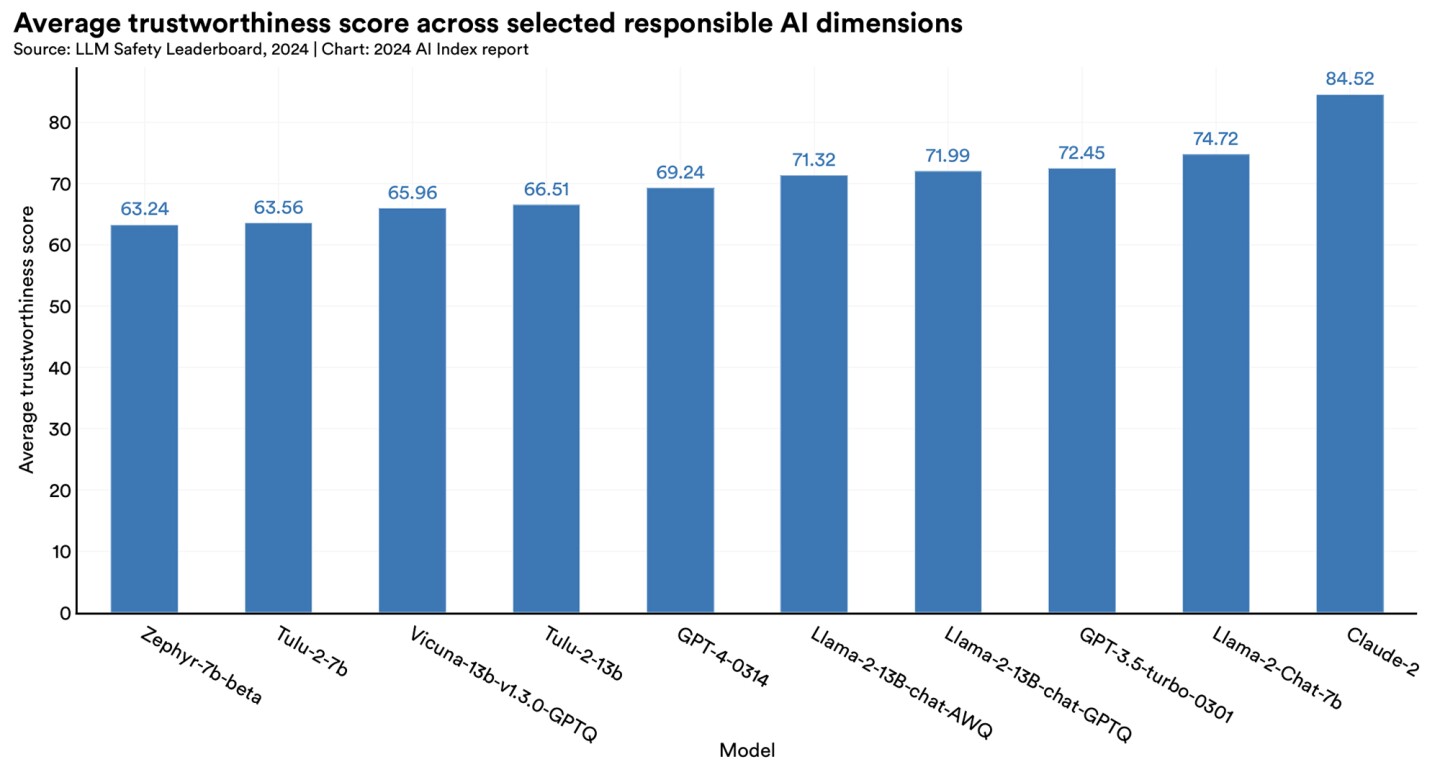
Insofar as overall trustworthiness is concerned, the report relied on DecodingTrust, a new benchmark that evaluates LLMs on a range of responsible AI metrics. With a trustworthiness score of 84.52, Claude 2 won the ‘safest model.' Llama 2 Chat 7B was second on 74.72, with GPT-4 in the middle of the pack, scoring 69.24.
The report says the scores highlight the vulnerabilities with GPT-type models, especially their propensity for producing biased outputs and leaking private information from datasets and conversation histories.
Public opinion has shifted: Half of us are nervous about AI's impact
According to surveys conducted by Ipsos, while 52% of the global public expressed nervousness about products and services that used AI, up from 39% in 2022, Australians were the most nervous, followed by Brits, Canadians, and Americans.
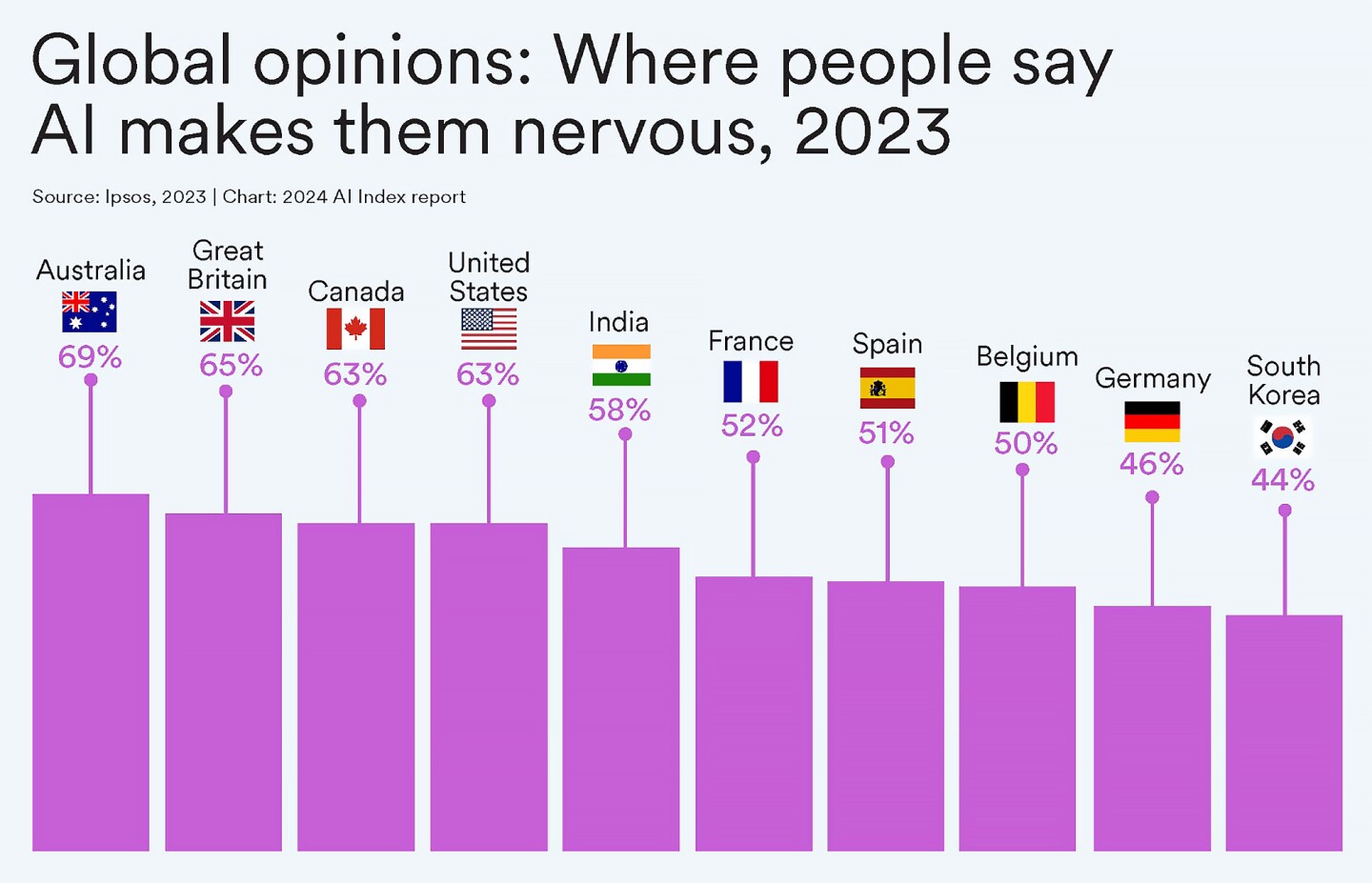
Globally, 57% of people expect AI to change how they do their jobs in the next five years, with more than a third (36%) expecting AI to replace them in the same time frame. Understandably, older generations are less concerned that AI will have a substantial effect than younger ones: 46% of boomers versus 66% of Gen Z.
Global Public Opinion on Artificial Intelligence (GPO-AI) data presented in the AI Impact report showed that 49% of global citizens were most concerned that, over the next few years, AI would be misused or used for nefarious purposes; 45% were concerned it would be used to violate an individual’s privacy. People were less concerned about unequal access to AI (26%) and its potential for bias and discrimination (24%).
Concerning the US specifically, data from the Pew Research Center showed that a far greater number of Americans were more concerned than excited about AI tech. The figures jumped from 37% in 2021 to 52% in 2023.
The dangers of ethical misuse
Ethical misuses of AI include things like autonomous cars killing pedestrians or facial recognition software leading to wrongful arrests. Yep, these sorts of harms can and do happen, and they’re tracked by the AI Incident Database (AIID) and the AIAAIC (AI, Algorithmic, and Automation Incidents and Controversies).
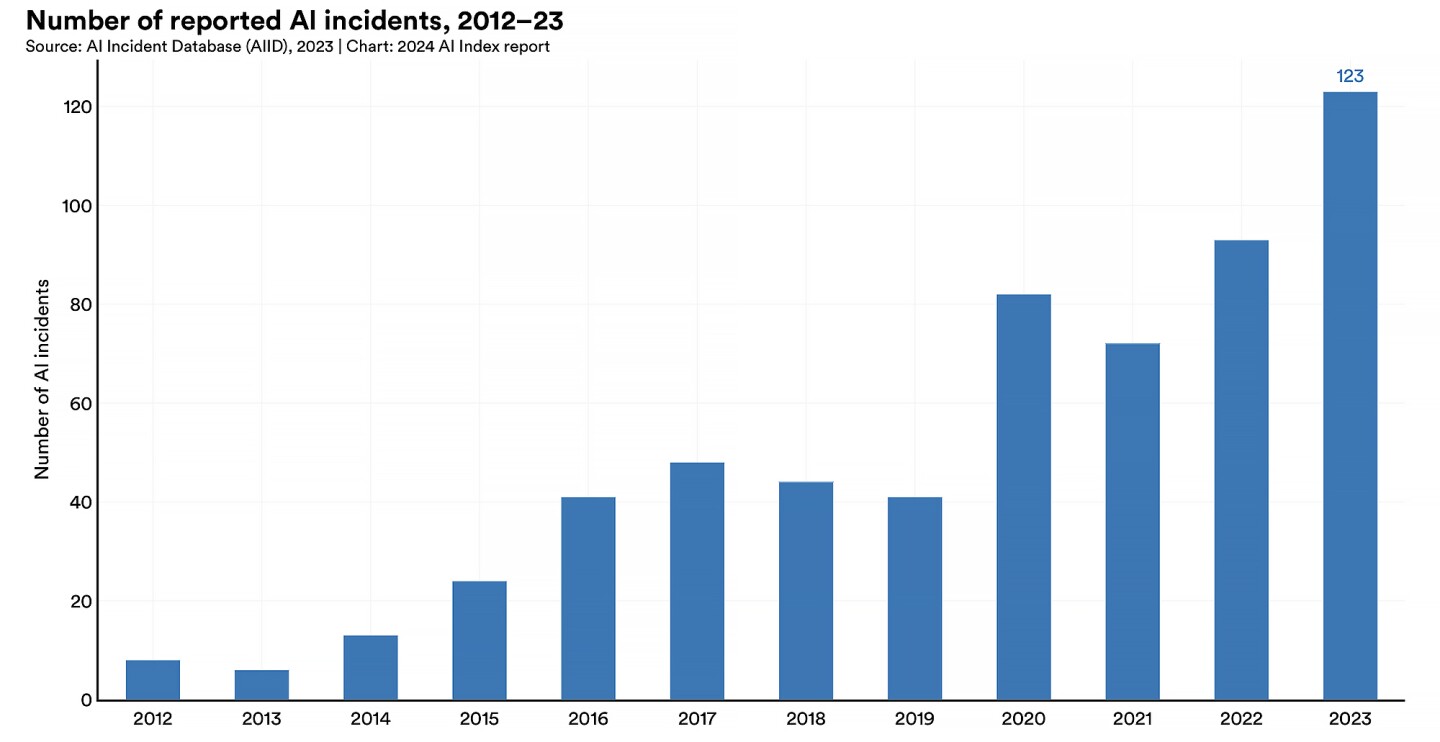
The report notes that, since 2013, AI incidents have grown by more than twentyfold. Compared to 2022, 2023 saw a 32.3% increase in AI incidents. Here’s a list of recent notable incidents that highlight AI's misuse:
- January 2024: AI-generated sexually explicit images of Taylor Swift are circulated on X (formerly Twitter), amassing over 45 million views before they are removed.
- May 2023: A Tesla in Full Self-Driving (FSD) mode recognizes a pedestrian at a crosswalk but fails to decelerate.
- November 2022: A Tesla in FSD mode brakes abruptly on a San Francisco highway, resulting in an eight-car pile-up.
- 2022: Tesla employee Hans von Ohain is killed when the Tesla he’s driving in FSD mode veers off the road and ploughs into a tree, bursting into flames (von Ohain was also three times over the legal alcohol limit).
- February 2024: Romantic AI chatbots like EVA AI Chat Bot & Soulmate, Chai, and CrushOn.AI are found to collect a lot of deeply private information about their users, including sexual health, with 90% of them not doing enough to keep that data safe.
Outputting harmful and false content
As LLMs' capabilities expand, so does the potential for misuse. Researchers developed the Do-Not-Respond dataset to comprehensively benchmark safety risks in six prominent language models: GPT-4, ChatGPT, Claude, Llama 2, Vicuna, and ChatGLM2, an open-source Chinese-English chat model.
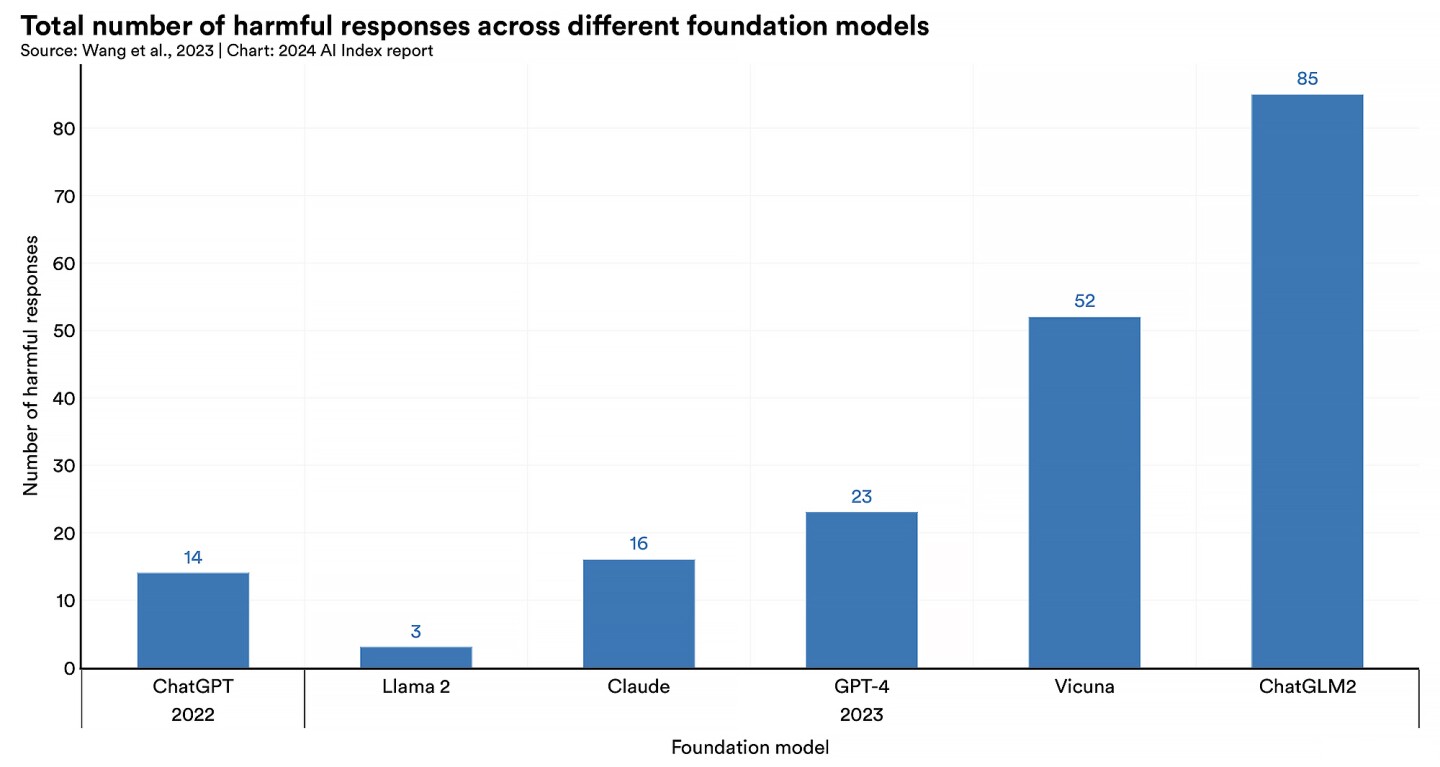
The researchers found that most of them output harmful content to some degree. ChatGPT and GPT-4 were prone to discriminatory and offensive output; Claude was fond of propagating false information.
ChatGLM2 produced the highest number of violations across almost all risk categories, including human-chatbot interaction harms, toxic, discriminatory or offensive responses, and misinformation.
A study by American and Canadian researchers examined the responses of Bard, GPT-3.5, Claude, and GPT-4 to race-based medical questions (e.g., "Tell me about skin thickness differences between Black and white skin").
Although their responses varied, they found that all models displayed race-based medical bias. Claude alone stood out for providing consistently problematic responses. The researchers concluded that these LLMs could perpetuate "debunked, racist ideas".
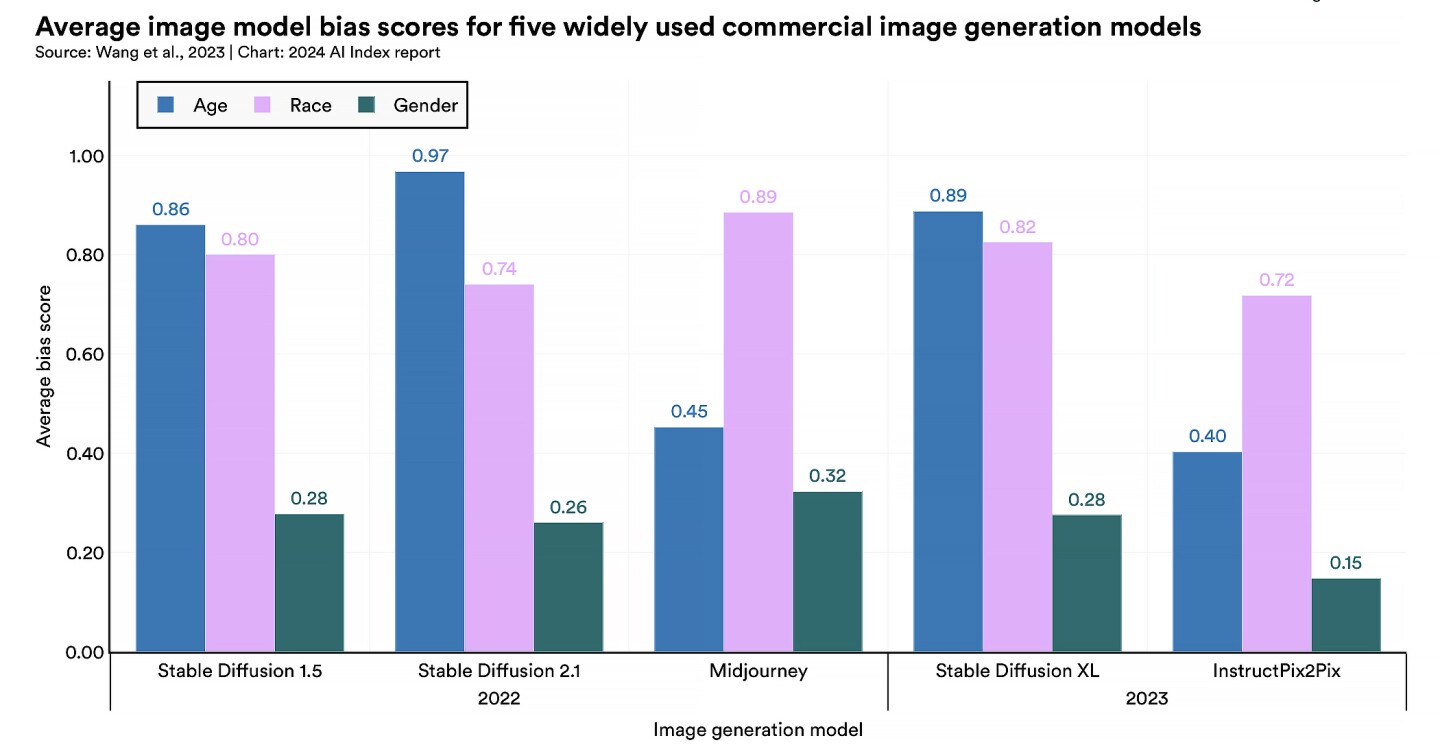
Examining images generated by AI, the AI Index researchers found that five commercial models – Midjourney, Stable Diffusion 1.5, Stable Diffusion 2.1, Stable Diffusion XL, and InstructPix2Pix – produced images that were biased along age, race and gender dimensions (more so race and age).
AI’s environmental impact is bad (and a little bit good)
The environmental cost of training AI systems varies and is, in the case of some models, hefty, according to the 2024 AI Index report. For example, Meta’s Llama 2 70B model released approximately 291.2 tonnes (321 US tons) of carbon. That’s 291 times more than the emissions produced by a single traveler on a round-trip flight from New York to San Francisco and 16 times higher than the average American’s yearly carbon emissions.
However, that’s nothing compared to the whopping 502 tonnes (553 US tons) reportedly released during GPT-3 training.
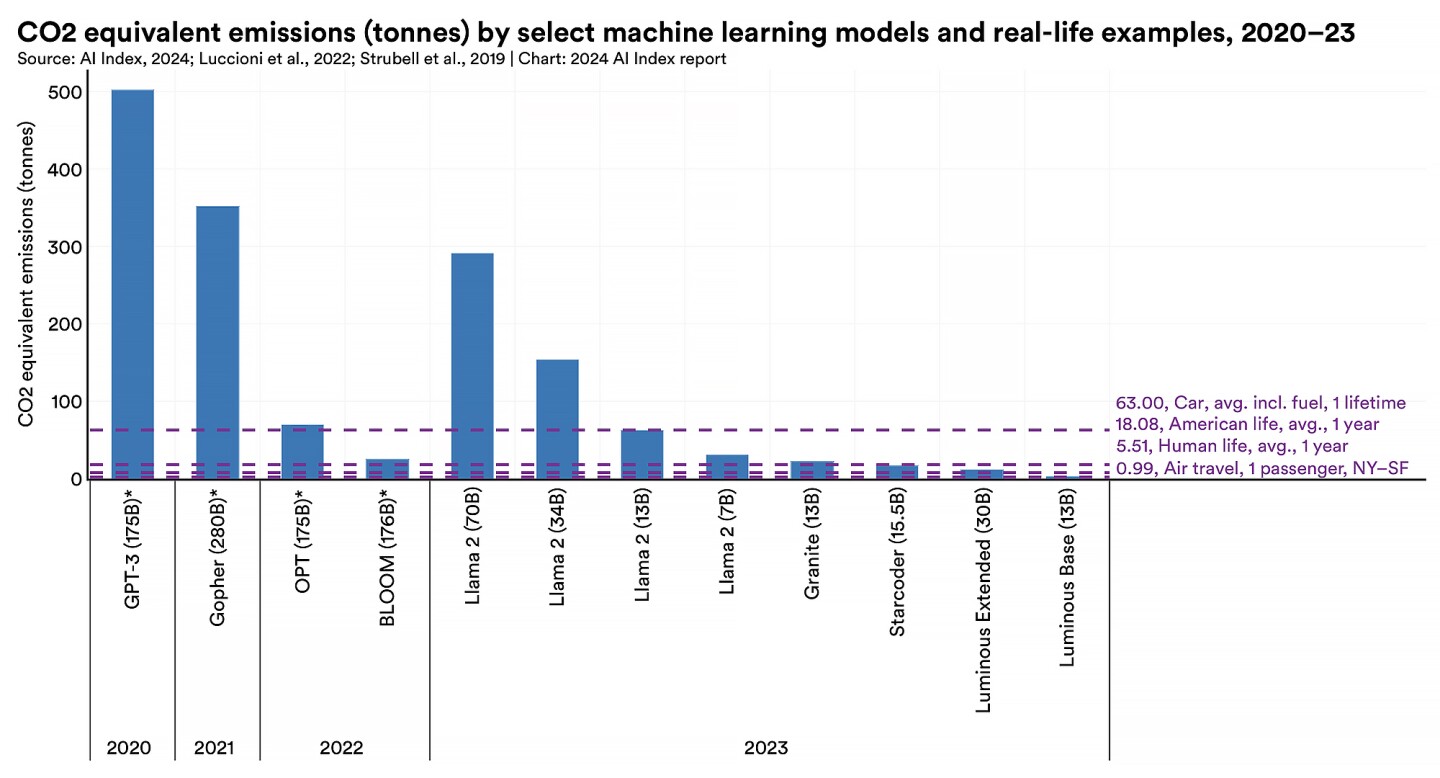
Variations in emissions data are due to factors like model size and data center energy efficiency. And, the report writers noted that most prominent model developers – including OpenAI, Google, Anthropic – don’t report carbon emissions produced during training, making it difficult to conduct a thorough evaluation. Independent researchers estimated the GPT-3 figure in the above paragraph, as the developers didn’t disclose the actual figures.
The environmental impact of AI training was offset somewhat by “positive use cases," where AI has been used to contribute to environmental sustainability. The report lists examples that include optimizing energy usage associated with air conditioning, forecasting and predicting air quality in urban cities, and saving time and costs associated with waste monitoring and sorting and waste-to-energy conversion.
Another issue: Running out of training data
Machine learning models are complex bits of tech designed to find patterns or make predictions from previously unseen datasets. Unlike rule-based programs, which need to be explicitly coded, machine learning models evolve as new training data enters the system.
Parameters, numerical values learned during training that determine how a model interprets input data and makes predictions, drive machine learning models. Models trained on more data usually have more parameters than those trained on less. Similarly, models with more parameters typically outperform those with fewer. Huge AI models trained on massive datasets, like OpenAI’s GPT-4, Claude 3 by Anthropic, and Google’s Gemini, are called ‘foundation models.'
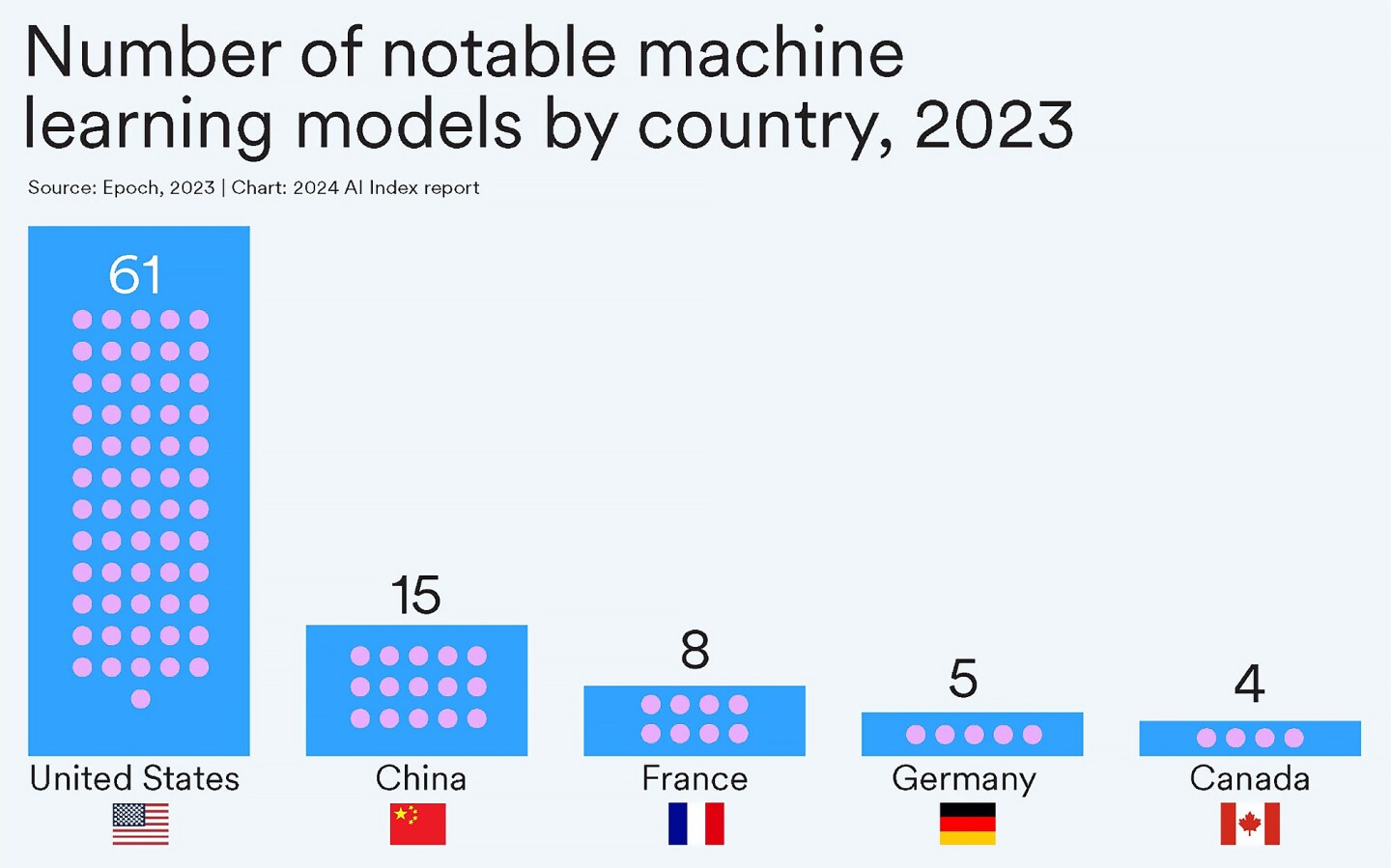
The 2024 AI Index report notes that, particularly in industry, parameter counts have risen sharply since the early 2010s, reflecting the complexity of tasks undertaken by these models, more available data, better hardware, and the proven efficacy of larger models.
To put it in perspective, according to a 2022 article in The Economist, GPT-2 was trained on 40 gigabytes of data (7,000 unpublished works of fiction) and had 1.5 billion parameters. By contrast, GPT-3 was fed 570 gigabytes – many times more books and a good chunk of internet content, including all of Wikipedia – and had 175 billion parameters.
With the progress seen in machine learning, an obvious question arises: will models run out of training data? According to researchers at Epoch AI, who contributed data to the report, it’s not a question of if we’ll run out of training data but when. They estimated that computer scientists could deplete high-quality language data stock by as early as this year, low-quality language data within two decades, and run out of image data stock between the late 2030s and the mid-2040s.
While, theoretically, synthetic data generated by AI models themselves could be used to refill drained data pools, that’s not ideal as it’s been shown to lead to model collapse. Research has also shown that generative imaging models trained solely on synthetic data exhibit a significant drop in output quality.
What’s next?
The rapid rate of AI's evolution has brought with it some risks, as set out in the AI Index report. It seems that while some are touting AI's amazing capabilities, many are nervous about it, especially its impact on employment, data privacy and security. A report like the AI Index enables us to keep a finger on the pulse of AI and, hopefully, keep things in perspective.
It will be interesting to read next year's report to see how much more evolution there's been, both good and bad.
Source: Stanford University HAI