With all the stats and analysis getting batted around, you could be forgiven for thinking that modern sport is not about the grand battles between opposing players and teams but rather an elaborate exercise in data modelling. An entire industry is forming around predictions and tracking in sports, on the one hand to understand and on the other to compete better. Now Disney Research has released two new studies that help both of these along. One study analyzed soccer player and ball movement patterns to detect and visualize team formations, while the other built models that could accurately predict whether a basketball player will pass or shoot in a given game situation.
The automated, data-driven methods described in both studies are meant to help players and coaches scout opposition teams and plan for specific game situations, as much of elite-level sport depends on anticipating other players' behavior and identifying optimal strategies.
The basketball research took player tracking data from every half-court possession (where at least four players from each team have been in half court for at least four seconds) in 630 basketball games in the 2012-13 NBA season. The model considered distances between the ball handler and the basket and his teammates and opponents, as well as the amount of time he has held the ball. It did not, however, account for scoreline, fatigue, time remaining on the clock, or even player trajectories (i.e., their movement over the previous few seconds).
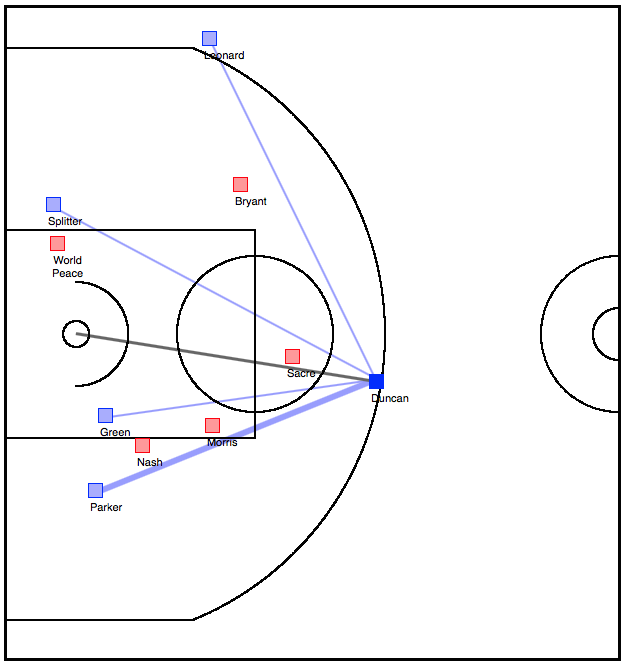
Despite the simplicity of the model, the researchers found that it makes predictions with a high degree of accuracy – especially after incorporating player tendencies in certain locations, as identified by the software in analyzing the data. Most promisingly, the model's findings of when a player is likely to shoot, hold the ball, or pass correspond with known intuitions of basketball gameplay.
The soccer study had to approach the questions of prediction and automated analysis differently. "In basketball, you have a lot of events and outcomes," explains Patrick Lucey, an associate research scientist at Disney Research Pittsburgh. The 24-second shot clock ensures that possession changes frequently, and scores tally quickly, which provides a lot of data points for evaluating individual play. But goals are rare in soccer – seldom more than one or two in a game. "It means you have to understand behaviors in the absence of scoring," Lucey says.
Moreover, focusing on the movements and behaviors of individual players can be misleading. They might change positions during the game, perhaps switching from the left wing to the right or from central midfield to attack. Players are cogs in a larger machine, and even the standouts like Lionel Messi and Cristiano Ronaldo fill a role. That contextual information is what the Disney researchers sought to identify from the data.
Their model analyzed 400 million player and ball tracking data points across an entire season in a professional league to plot a "role-based" representation of teams. It visualizes team formations and identifies each player's relative role at each frame through a method called minimum entropy data partitioning. This involves partitioning the set of player positions into clusters – each role is defined as occupying a particular region of the pitch, relative to the others (meaning that the exact coordinates may change), and players shift between them dynamically depending on tactics and circumstance.
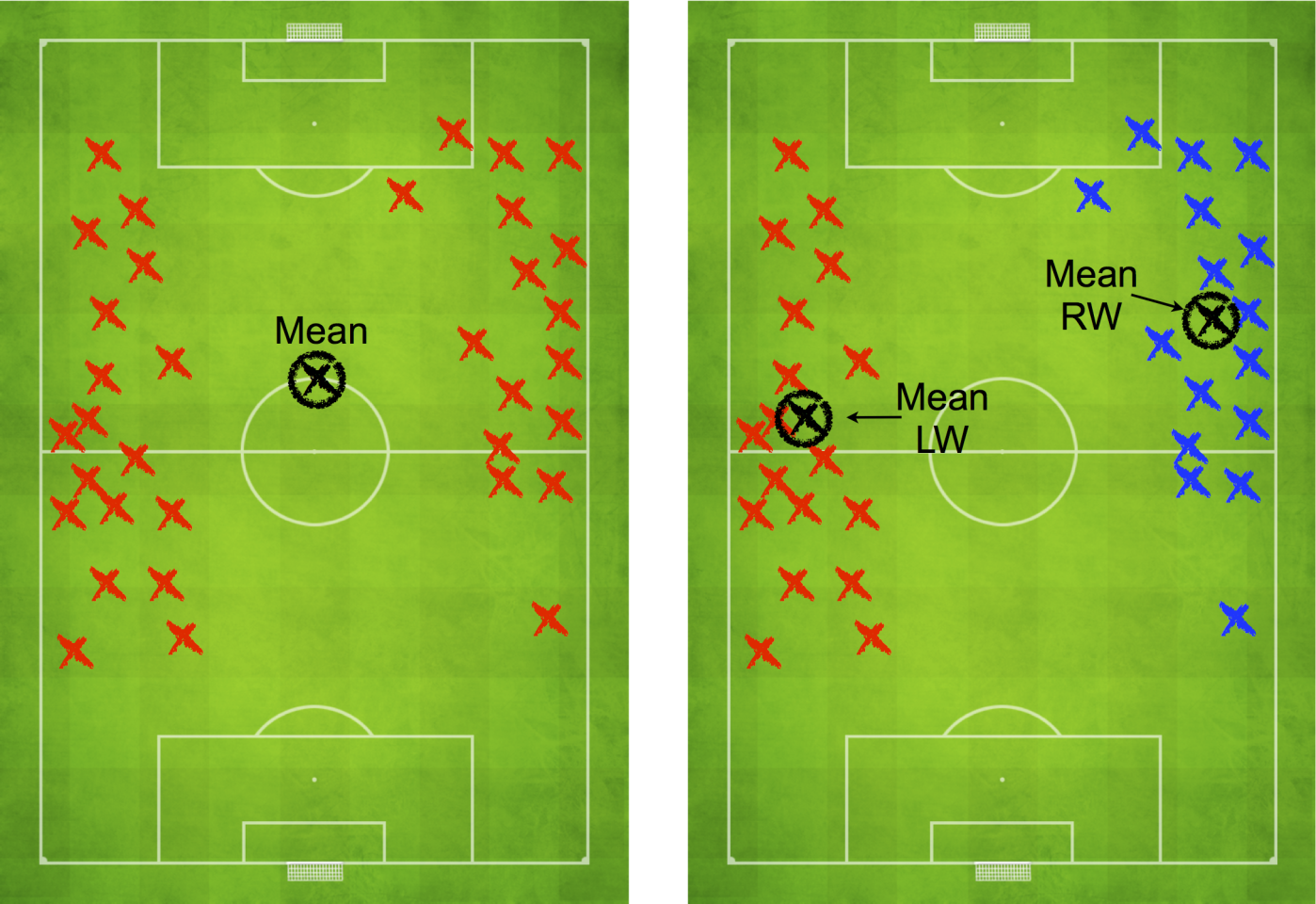
This kind of large-scale data mining could help in analyzing individual player behaviors, as it adds important context to the statistics. But its main value will be in a deeper exploration of team styles – strategic patterns and tendencies – to see just how great teams like Barcelona dominate so effortlessly and where other teams might improve their tactics and movement throughout a match. And that sort of deep dive is exactly what the researchers plan to do next.
Papers describing both the large-scale soccer analysis and basketball play prediction studies are available on the Disney Research website. Both studies are being presented this week at the International Conference on Data Mining in Shenzhen, China.
Source: Disney Research