Having taken on everyone from chess grandmasters to chefs, computers are further exploring their artistic side with computer scientists demonstrating how artificial neural networks can create works of art reminiscent of William Blake on opium. The surreal images produced by a technique called "Inceptionism" are part of a process to better understand how such networks operate and how to improve them.
Artificial neural networks are a form of artificial intelligence based on biological neural networks, such as the central nervous system. Unlike more conventional software that works according to rigidly defined rules, artificial neural networks are trained by being shown millions of training examples and having their network parameters slowing adjusted until they return the desired results.
The network is made up of nodes that act like interconnected neurons. These allow computers to handle large numbers of data inputs, the nature of which are either unknown or approximate. The idea is that these nodes are set in stacked layers with each layer handling data of increasing complexity, which they weigh according to their instructions. These layers have the ability to learn and become better at detecting desired patterns as they are fed more data over time.
This ability has made artificial neural networks very valuable in areas like image classification and speech recognition. For example, image recognition systems using artificial neural networks can handle images in the real-world chaos of unpredictable lighting, angles, colors, and backgrounds. Instead of following strict rules for sorting out what in a picture is a banana, a neural network can operate by using millions of examples to learn how to find simple things like edges, combine these into more complex shapes, and then combine these to identify various objects – one of which could be a banana.
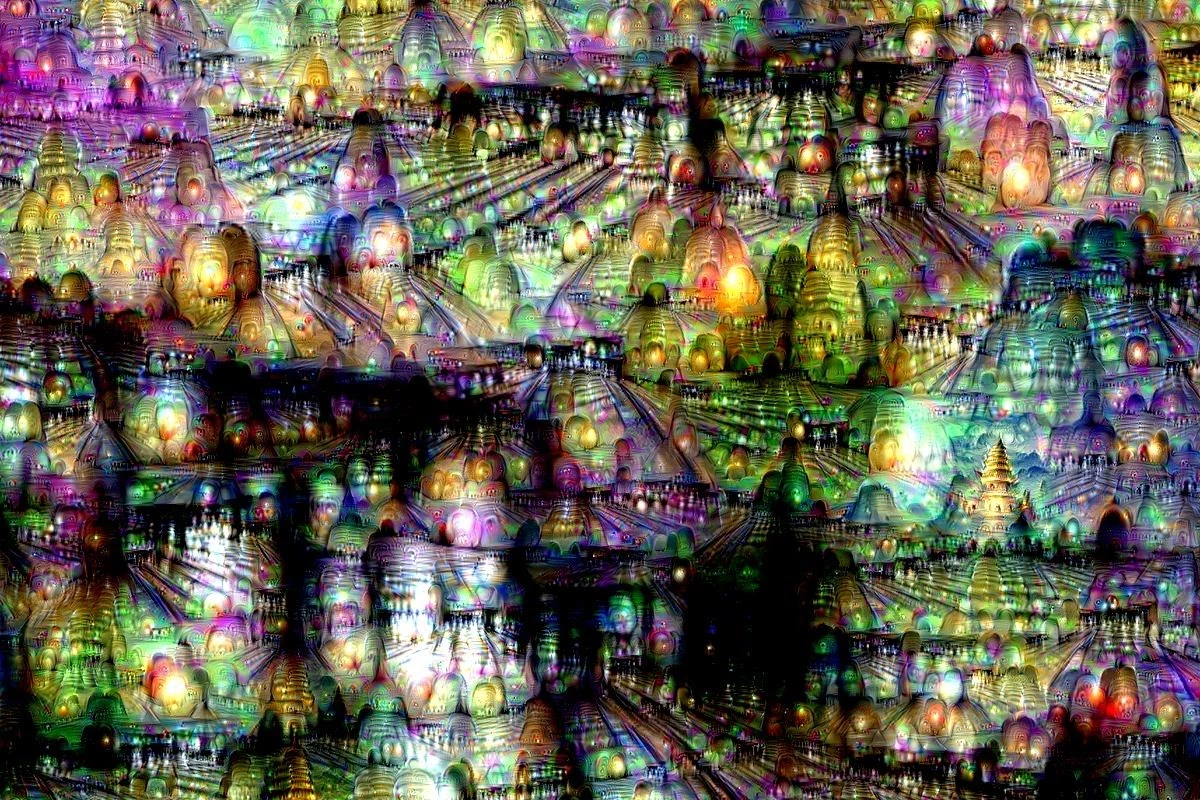
Unfortunately, according to Google Research, the very thing that makes artificial neural networks so effective also makes them very difficult to understand. The mathematical models they use may be very well known, but after data has passed through up to 30 layers of the network, exactly what is going on isn't entirely clear. The input goes into the first layer and the end result is outputted from the last, but what happens in between is not always certain.
To better understand this process, the Google Research team is reversing the process as part of Inceptionism. Instead of looking at an image and seeking a particular object, the network was told to use its knowledge and rules to look for things that could be an object, such as a banana, and enhancing it until it resembles what the network regards as a banana.
What the researchers discovered was that the networks didn't just have the ability to recognize objects, but once they've been properly tutored, they have enough information to generate images statistically with a high degree of accuracy – not to mention what seems like a Daliesque imagination.
The networks can take a seemingly plain image, such as a landscape, and turn into a skyline of towers, pagodas, and cupola-capped palaces. Cloudscapes become a phantasmagoria of animals and birds, trees become strange castles, and the Seattle Space Needle becomes a gothic spire covered with weird living shapes like something out of a Lovecraft novel.
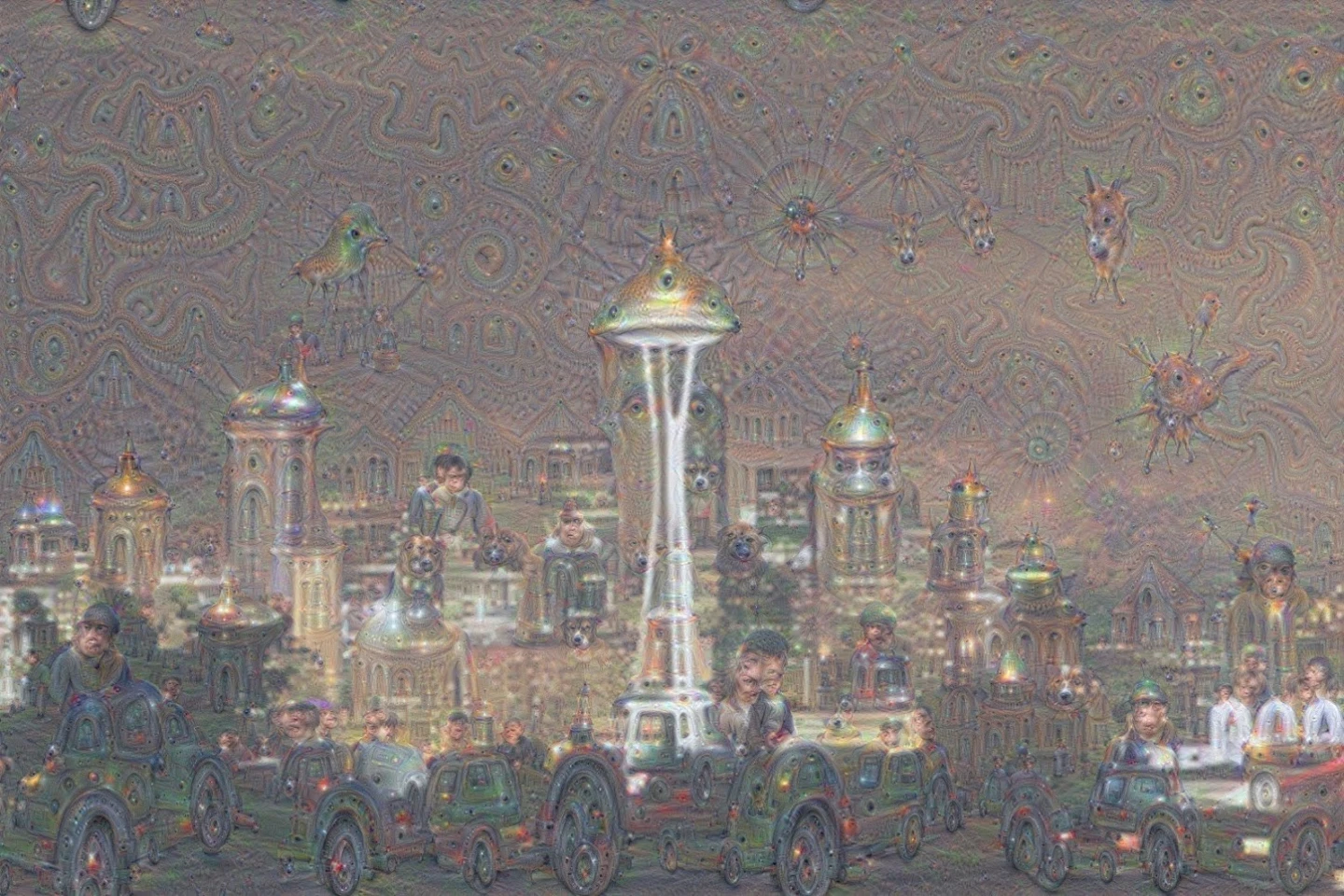
According to the researchers, the point of all of this is that by selecting various layers, letting the network choose what features to enhance, and changing the orders given to weight the results, the generated images can give the team a better understanding of what is going on.
For example, each layer represents a different layer of complexity, so the final image reflects this. Lower levels address simple things like edges and create strokes and ornament-like patterns, while higher layers produce results of greater complexity, and by asking the network, the researchers can create a feedback loop, which could turn a patch of wispy cloud into a full-blown animal.
The team says that Inceptionism provides important insights into how the networks learn and come to their conclusions by studying the images the networks create and solving problems that arise. In identifying images, the networks are expected to figure out key features, like the tines on a fork. However, machine logic can produce peculiarities. One example is the network's attempt to identify a dumbbell. A human being regards it as a simple piece of exercise equipment, but the images used to train the network tend to show dumbbells held in hands, so when it generates an image of one in Inceptionism, they invariably depict a dumbbell as having an arm attached.
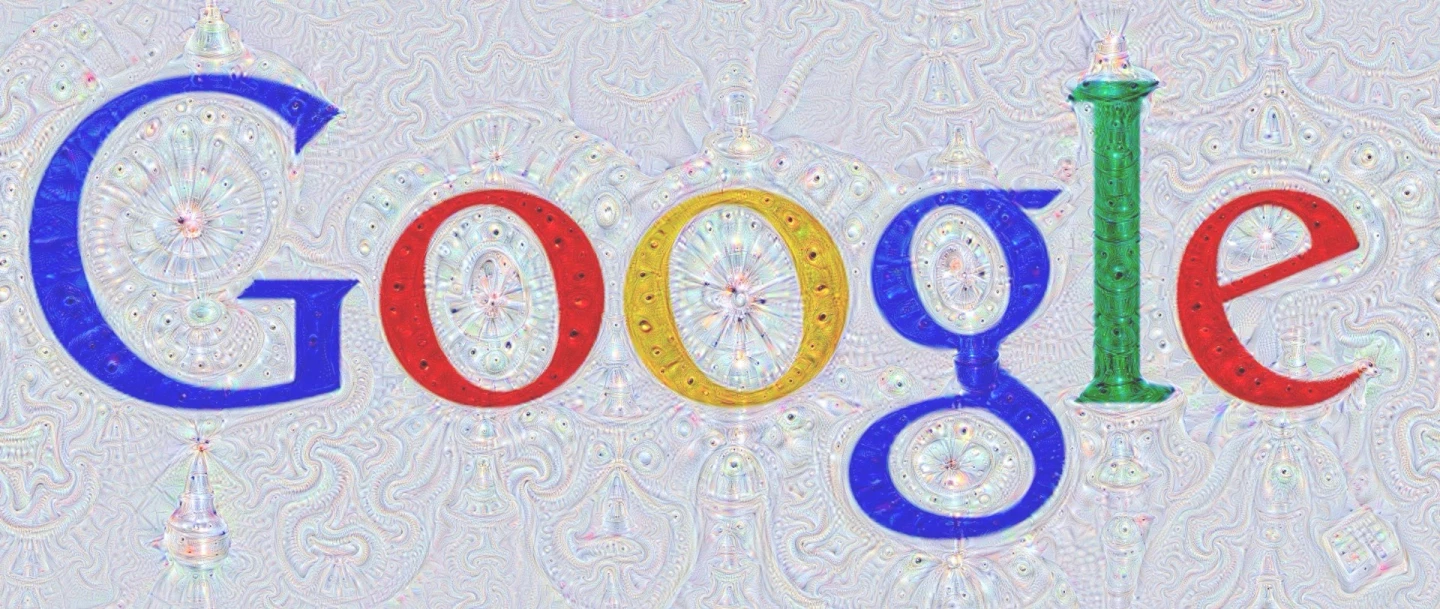
In addition to this, the researchers can study how the networks transform certain objects into new images. The training of the network introduces biases, so rocks and trees become buildings, horizons turn into exotic skylines, and leaves become birds and insects. In addition, Inceptionism can work a bit like fractals with zooming in on a result producing more images, so the network is creating new images from images it's already created.
The Google research team sees Inceptionism as having a number of applications beyond the psychedelic. In addition to a better understanding of artificial neural networks, the technique can help to improve network architecture and as a check on network learning. It may even one day become a new tool for artists and even provide new insights into the creative process.
Source: Google Research