For all their hype, self-driving cars are still quite clueless at many tasks that are simple for human drivers, like recognizing a sidewalk or a traffic light. Scientists at the Computer Vision Center in Barcelona have now come to the rescue with Synthia, a virtual city simulation that can train driving AIs to recognize and handle all sorts of obstacles and situations, even in rain or deep snow.
Real data, real headaches
If you believe Elon Musk, you'll think that self-driving cars will one day become so safe that they will replace human drivers altogether. That may well be true, but, even tough Tesla is pushing the boundaries with its semi-autonomous features, it will still be a while before you can simply put your feet on the dashboard and let your Model S drive you to work.
Neural networks, which are a key component of driving AIs, are being trained on an extensive set of real-world images and videos to accurately recognize different "classes" of objects, such as cars, pedestrians, road signs, and so on. Using these classes, the software can then try to interpret real-time input from the car's cameras and decide whether to steer, brake, or signal a lane change.
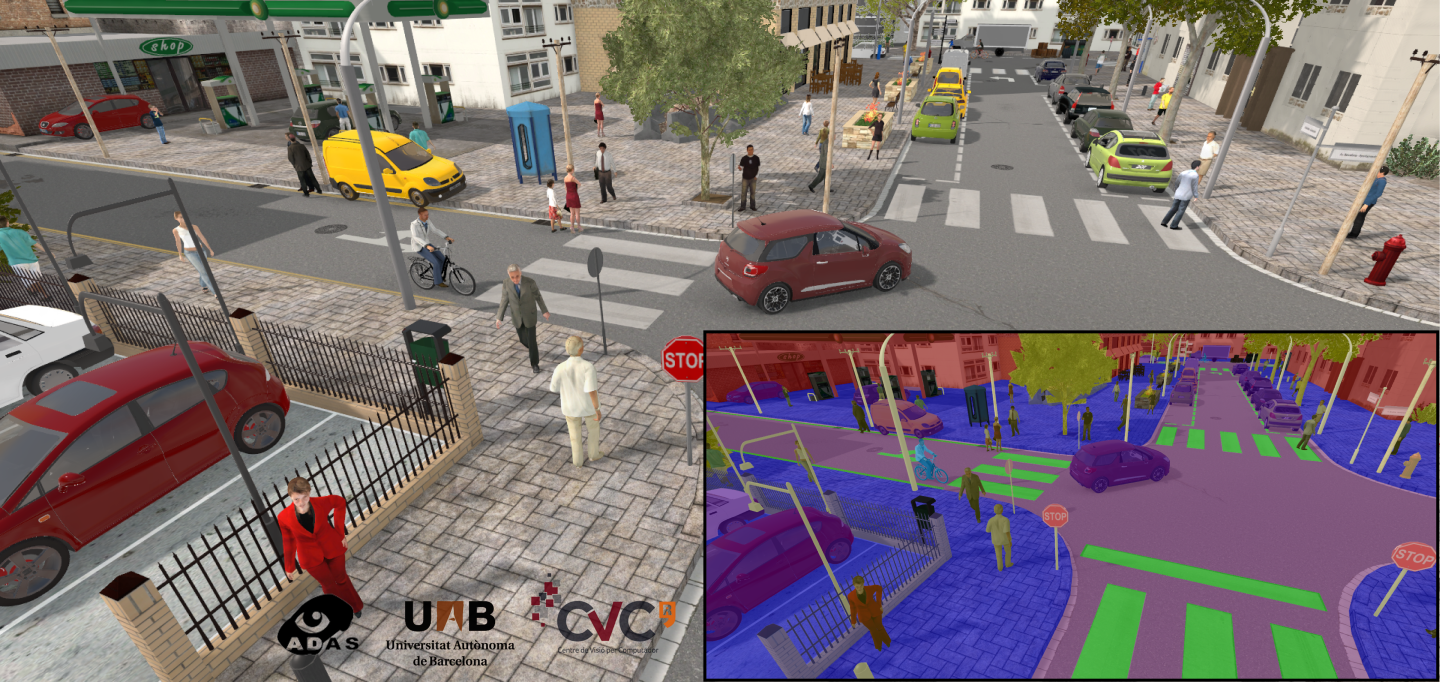
But while driving AIs can collect plenty of data on common situations like driving on the freeway, which is relatively easy in AI terms, the software has a much harder time trying to handle what engineers call "corner cases." These are events that happen rarely – such as car accidents, ambulances responding to an emergency, or maneuvering construction vehicles – and are therefore pose difficulties in collecting a large enough sample of real-world data with which to train self-driving software.
Even worse, the images used to train the neural networks must be annotated manually: that is to say, someone needs to painstakingly go through each picture and label different elements on a pixel by pixel level, separating drivable road from sidewalk, or a pedestrian from a road sign. This is what Daimler did with the CityScapes project, manually annotating more than 20,000 images and separating objects into 30 different classes. Mobileye, which provides the software used by Tesla's autopilot system, currently employs over 600 people to manually annotate images and is shooting for 1,000 by the end of the year.
Clearly, this is has been an expensive problem to solve – and it still doesn't address the problem of corner cases.
Roaming a virtual world
German Ros and his team at the Computer Vision Center in Barcelona have now found a way to correctly annotate images automatically and teach driving AIs how to behave even in the most unusual situations imaginable, all from inside a video game.
Using the popular Unity engine, the researchers started by creating a realistic simulation of a not only a city and its surroundings, complete with pedestrians, cyclists and poorly parked buses, but also a complex weather system that includes rain, snow, and seasons. They then "built" a virtual car inside the simulation, chose specific positioning and orientation for the car's autopilot cameras, and let the car roam the virtual world, shooting video and pictures from the camera's vantage point.
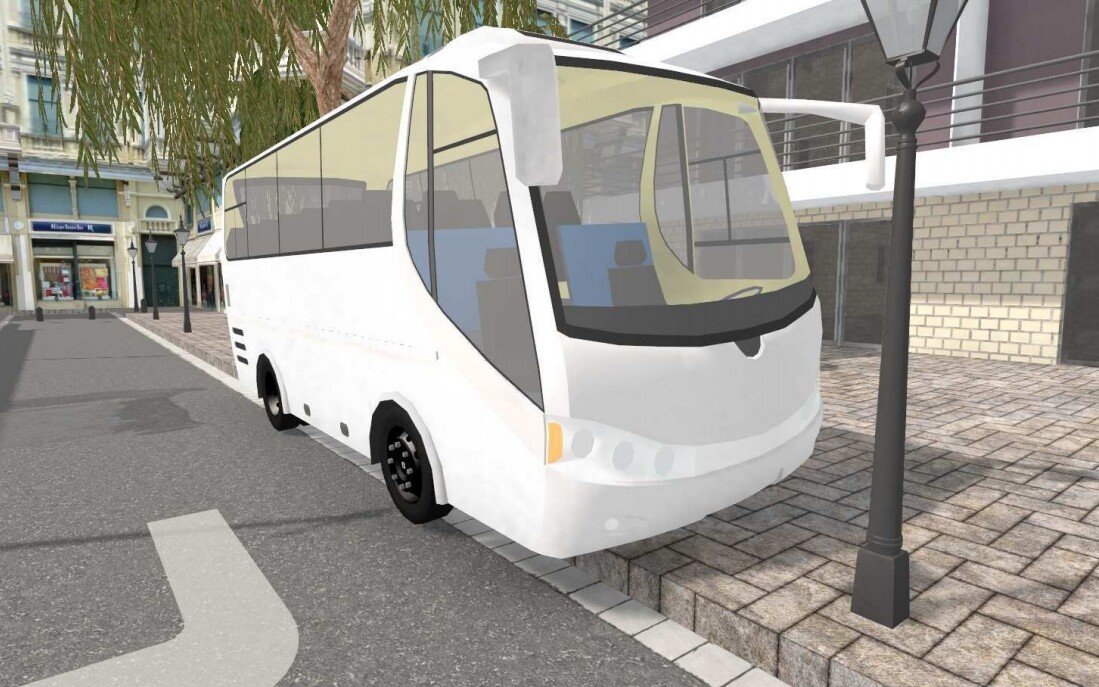
Because the software can identify with complete accuracy what the virtual cameras have captured, the system can generate a very large collection of realistic, impeccably annotated pictures and video which the researchers have dubbed Synthia (Synthetic collection of Imagery and Annotations of urban scenario).
Along real-world images, the data can then be fed to a neural network to train it, eliminating the need for huge amounts of time- and labor-intensive manual annotations, and even helping driving software recognize some of the objects they usually have a harder time with.
"AIs are becoming very good at recognizing objects such as pedestrians or vehicles," Ros tells us. "However, the boundaries of sidewalks and the recognition of traffic lights are still very challenging. Sidewalks change dramatically from country to country, from city to town. Thanks to Synthia we can produce corner cases with no risk, and focus on those."
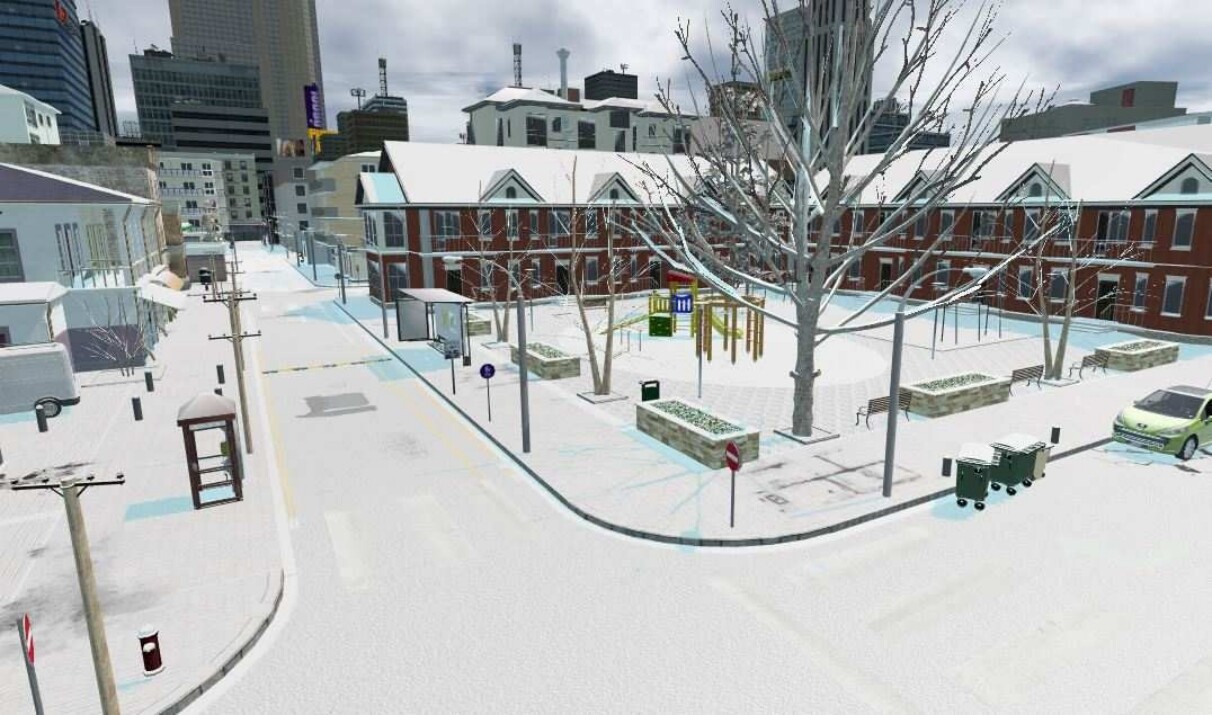
The researchers collected more than 213,000 virtual images and video sequences, and sought to see whether training neural networks on a combination of real and virtual images would improve the software's recognition capabilities on real-world images. The mix they used, Ros tells us, was typically two percent or less of real-world, manually annotated pictures, with the remainder from the Synthia database.
Using as a baseline eight different algorithms that processed low resolution (240 by 180 pixels) images, the team saw that adding the synthetic images to the manually annotated ones improved the image recognition capabilities substantially. When trying to classify small areas of those images into one of 11 classes, the average success rate jumped from about 45 to around 55 percent. Commercial driving software uses higher-quality source images, so their accuracy will be higher, but Ros says the analysis is still a clear indication of Synthia's efficacy.
The scientists are releasing all the data produced by Synthia with a public license for non-commercial use to get feedback and further improve the platform. Ros also tells us there are commercial agreements in place with yet to be announced car manufacturers to adapt the camera configuration of Synthia's "virtual car" to match the manufacturers' specifications.
The video below shows the system in action.
Sources: Computer Vision Center, The Computer Vision Foundation (PDF)