For deaf and hard-of-hearing people, voice recognition technology like Alexa and Siri can be a barrier to effective communication. Researchers have used AI to develop a tool that converts sign language to text, potentially increasing inclusivity and accessibility for the deaf community.
Translating sign language requires a precise understanding of a signer’s pose to generate an accurate textual transcription. Researchers at the Barcelona Supercomputing Center (BSC) and the Universitat Politècnica de Catalunya (UPC) have used AI to develop a tool for improving sign language translation, an important step towards allowing deaf and hard-of-hearing people to interact with technology and access digital services designed for use with spoken languages.
The researchers used a transformer-style machine-learning model, similar to those behind other AI tools like ChatGPT. Transformers are useful for two main reasons. One, these models are particularly good at learning how to apply context, due to the self-attention mechanism present in the architecture – self-attention is how a neural network contextualizes words by looking at other words in the body of a text. And two, they allow much faster throughput when learning from training examples, enabling more training data to be used at a given time.
Here, the training dataset came from How2Sign, a publicly available large-scale, multimodal and multi-view dataset comprising 80 hours of instructional videos in American Sign Language with corresponding English transcripts.
“The new tool developed is an extension of a previous publication also by BSC and the UPC called How2Sign, where the data needed to train the models (more than 80 hours of videos where American Sign Language interpreters translate video tutorials such as cooking recipes or DIY tricks) were published,” said Laia Tarrés, lead author of the study. “With this data already available, the team has developed a new open-source software capable of learning the mapping between video and text.”
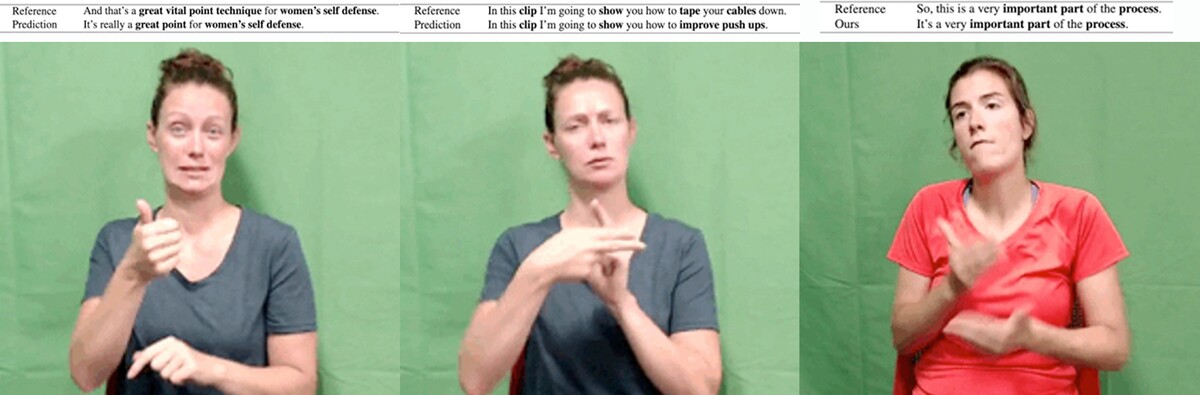
For the researchers, it was important to use videos of continuous signing rather than isolated signing, as it more realistically reflects how speakers naturally use a chain of words (concatenation) to construct sentences which can be crucial in determining a sentence’s meaning.
A challenge faced by the researchers was the variability and complexity of sign languages, which can be influenced by things such as the signer’s background, context, and appearance. To help in that regard, they pre-processed the data using Inflated 3D Networks (I3D), a method of video extraction that applies a 3D filter to videos, allowing spatiotemporal information to be taken directly from them.
The researchers found that text pre-processing also significantly improved sign-to-text translations. To pre-process the raw text, they converted it all to lowercase which reduced the vocabulary complexity.
Overall, they found that their model was able to produce meaningful translations, but was not perfect. “While our work has shown promising results, there is still room for improvement,” the researchers said.
With the model still in the experimental phase, the researchers will continue to work on creating a tool that allows deaf and hard-of-hearing people access to the same technologies as those without hearing loss.
“This open tool for automatic sign language translation is a valuable contribution to the scientific community focused on accessibility, and its publication represents a significant step towards the creation of more inclusive and accessible technology for all,” Tarrés said.
The study was published online at arXiv.
Source: Barcelona Supercomputing Center