If robots are going to work alongside humans, the machines are going to need to swallow their pride and learn to ask for help. At least, that’s the thinking of computer scientists at the University of Washington (UW), who are working on ways for robots to crowdsource their problems when learning new tasks. If successful, this approach points the way toward future robots that are capable of asking for assistance to speed up their learning when it comes to figuring out how to carry out household tasks.
Human environments are very unfriendly for robots. They prefer a tightly controlled environment where everything is predictable and objects are relatively easy to define. However, that’s exactly the opposite of the normal human environment, as anyone who’s cleaned up after an 11-year old can confirm.
The real world is extremely complicated, filled with variables, and routinely poses complex problems to robots tasked with carrying out the simplest of household chores. Stacking dishes in a cupboard is easy enough, but loading a dishwasher is surprisingly difficult. A robot can be taught to do it, but the number of different ways of carrying out such a task can make for a very long lesson.
A common approach for teaching a robot how to carry out a task is for it to imitate a person. Instead of telling the robot how to move and what to do, the machine watches the operator in one way or another, then follows the example. It works, but it’s time consuming and working in real-world situations requires a great deal of data to produce accurate models – more than a single teacher can provide.
The UW team calls their approach "goal-based imitation," and involves programming the robot to "infer" the goals of its operators by watching how the human carries out a task, such as building a model, and then calculating how to carry it out itself, taking into account time and difficulty, and determining what are the key factors in completing the task.
The UW team reasoned that instead of a single human teacher, the robot is programmed to consult an online community for input on the best way to accomplish its task. The robot requests data relevant to how to complete a task and the community returns a large set of alternatives for the robot to analyze.
The UW study was aimed at finding ways to help a robot to recreate simple models out of colored Lego blocks, such as a car, tree, turtle, or a snake. The goal was for the robot to create something that resembled the original, yet was simple enough for it to assemble.
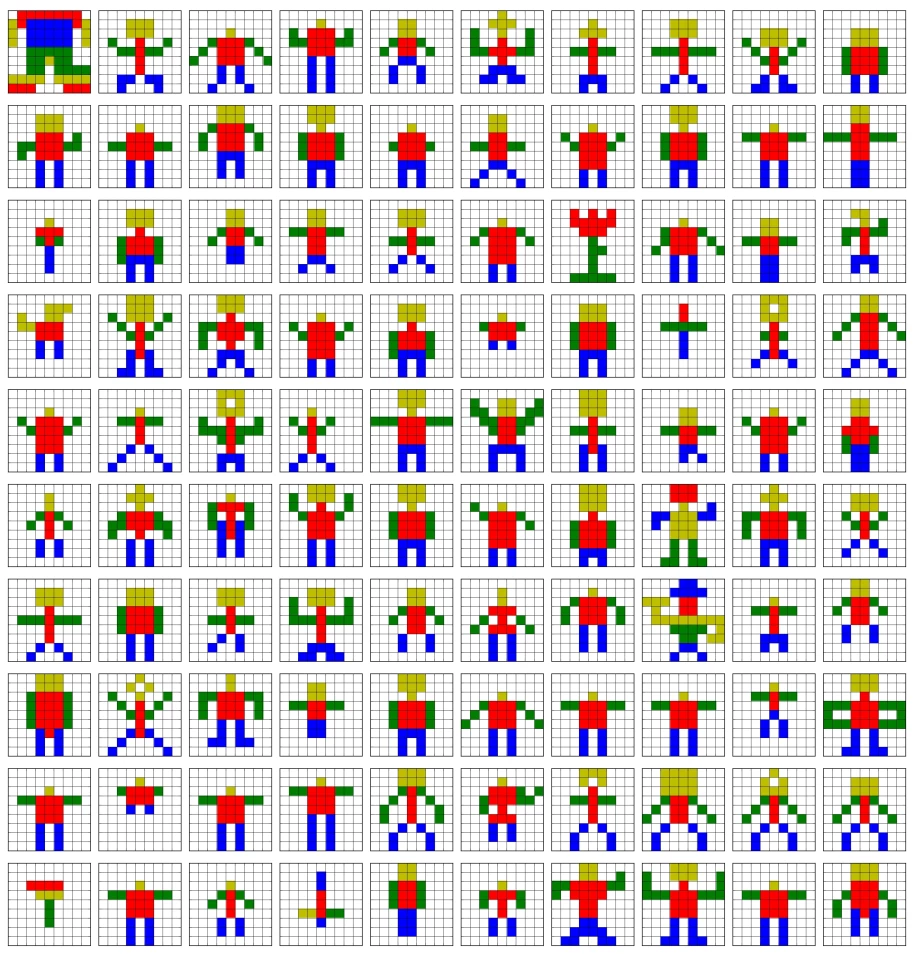
For the study, human participants built models of the object in question and the robot was tasked with replicating them. The robot couldn't manage this, so the team hired other participants online using the Amazon Mechanical Turk crowdsourcing site to create more models of the object in question. Based on the online community’s rating of the models, the robot chose the best ones for reconstruction. The outcome was a model that resembled the original, but was simpler and easier to construct.
"We’re trying to create a method for a robot to seek help from the whole world when it’s puzzled by something," says Rajesh Rao, associate professor of computer science and engineering and director of the Center for Sensorimotor Neural Engineering at UW. "This is a way to go beyond just one-on-one interaction between a human and a robot by also learning from other humans around the world."
The team presented its results at the 2014 Institute of Electrical and Electronics Engineers International Conference on Robotics and Automation in Hong Kong earlier this month and will report on their further research at the Conference on Human Computation and Crowdsourcing in Pittsburgh in November.
The following video shows the robot involved in the study.
Source: University of Washington