Across decades of development, scientists have been pursuing nuclear fusion technology through endless experiments, calculations and simulations, trying to find the optimal mix of conditions for atoms to fuse and continually release huge amounts of energy. The Alphabet-owned company DeepMind has now lent its considerable artificial intelligence know-how to the effort through a new partnership with Ecole Polytechnique Federale de Lausanne's (EPFL's) Swiss Plasma Center (SPC), where it has already proved its worth.
DeepMind has been making some impressive moves in the world of artificial intelligence over the past few years, beating the world's best players at Go, predicting rainfall with a high-degree of accuracy and even solving a 50-year scientific problem by predicting the 3D structures of unique proteins.
In harnessing the technology for nuclear fusion research, scientists hope to come up with ways to more successfully sustain streams of plasma, enabling more opportunities for critical fusion reactions to occur. The type of device used for these experiments at the SPC is known as a tokamak, which is a donut-shaped chamber that uses a powerful magnetic field to contain streams of super-hot plasma, in which hydrogen atoms fuse into one helium atom and release energy.
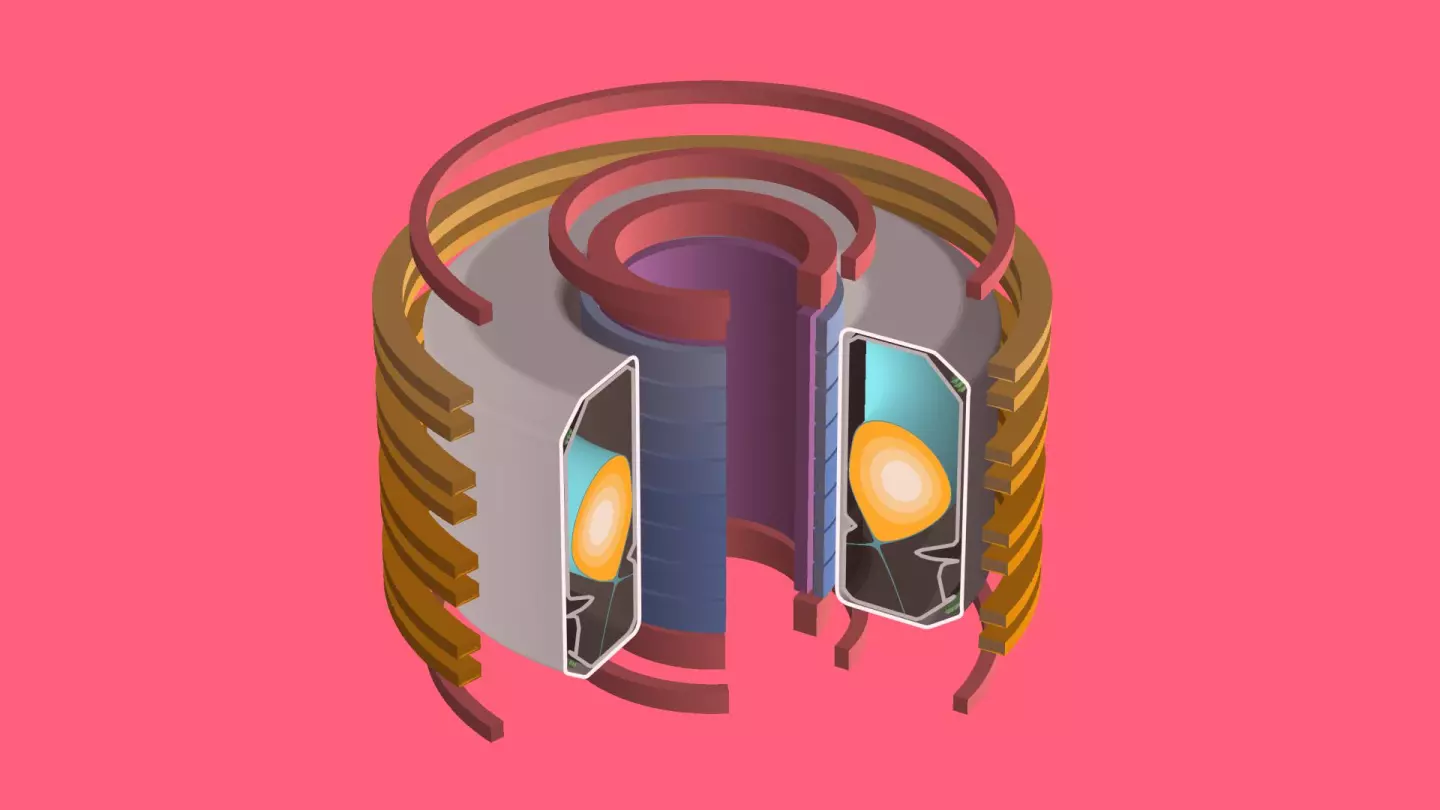
The SPC's tokamak is known as a variable-condition tokamak (TCV), in that it allows for experiments using plasma in different kinds of configurations. Researchers here are continually experimenting with new ways to control the plasma, so that it doesn't crash into the vessel walls and collapse.
"Our simulator is based on more than 20 years of research and is updated continuously," said Federico Felici, an SPC scientist. "But even so, lengthy calculations are still needed to determine the right value for each variable in the control system. That's where our joint research project with DeepMind comes in."
DeepMind developed a new AI algorithm that was trained on the SPC's simulator by having it attempt many different control strategies. In time, as it gained more experience through the simulations, the algorithm was able to calculate control strategies for producing requested plasma configurations. The team then tasked the algorithm to work in reverse, identifying the correct settings to generate a specific plasma configuration.
After training, the algorithm was tested on the real-world tokamak, where it was able to create and control a wide range of plasma shapes, including elongated and advanced shapes such as "negative triangularity" and "snowflake" configurations. One experiment involved maintaining two separate plasmas simultaneously.
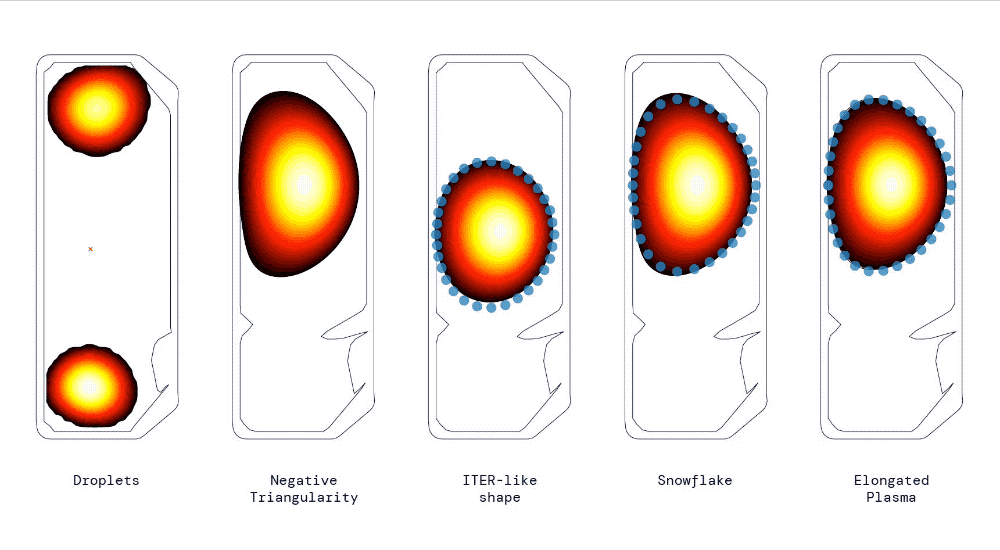
"Our team’s mission is to research a new generation of AI systems – closed-loop controllers – that can learn in complex dynamic environments completely from scratch," said Martin Riedmiller, control team lead at DeepMind. "Controlling a fusion plasma in the real world offers fantastic, albeit extremely challenging and complex, opportunities.”
The research was published in the journal Nature.
Source: EPFL