Researchers have used a machine learning algorithm to identify the top factors that can predict an adolescent’s risk of self-harm and attempting suicide. They say their model is more accurate than existing risk predictors and could be used to provide individualized care to vulnerable patients.
Adolescence is a critical formative period. Physical, emotional, and social changes can make adolescents vulnerable to mental health problems, including suicide attempts and self-harm. According to the Australian Institute of Health and Welfare (AIHW), suicide is the leading cause of death amongst Australians aged 15 to 24. In the US, the Centers for Disease Control and Prevention (CDC) lists it as the second leading cause for 10-to-14-year-olds.
The standard approach for predicting suicide or self-harm relies on past suicide or self-harm attempts as the only risk factor, which can be unreliable. Now, researchers led by the University of New South Wales Sydney have used machine learning (ML) to accurately identify the top factors that place adolescents at increased risk of suicide and self-harm.
“Sometimes we need to digest and process a lot of information that would be beyond the ability of the clinician,” said Ping-I Daniel Lin, corresponding author of the study. “That’s the reason we are tapping into machine learning algorithms.”
Data from 2,809 adolescents was extracted from the Longitudinal Study of Australian Children (LSAC), a nationally representative study that commenced in 2004. The adolescents were split into two age groups: 14-to-15-year-olds and 16-to-17-year-olds. The data came from questionnaires completed by the children, their carers and school teachers. Among the participants, 10.5% had reported an act of self-harm, and 5.2% reported attempting suicide at least once in the previous 12 months.
The researchers identified more than 4,000 potential risk factors from the data in areas such as mental health, physical health, relationships with others, and school and home environment. They used a random forest (RF) algorithm to identify which risk factors seen at age 14-15 were most predictive of suicide and self-harm attempts at 16-17.
RF is a supervised machine-learning algorithm made up of decision trees. It combines the output of multiple decision trees to reach a single result. The fundamental idea behind RF is that by combining many decision trees into a single model, predictions will be closer to the mark on average.
The predictive performance of the ML model was compared with an approach using only previous history of self-harm or suicide attempts as a predictor. The performance of each model was determined by evaluating the area under the curve (AUC), a performance metric that ranges from 0.5 (no better than random guessing) to 1.0 (perfect prediction). Generally, an AUC of 0.7 to 0.8 is considered acceptable at predicting risk, 0.8 to 0.9 excellent, and more than 9.0 is considered outstanding.
Forty-eight variables were used to train the RF model to predict self-harm, which showed fair predictive performance with an AUC of 0.740. In terms of predicting suicide attempts, the model, which was trained using 315 variables, achieved an AUC of 0.722.
For the self-harm model, the top variables identified included the Short Mood and Feelings Questionnaire (SMFQ), which assesses depression symptoms, Strengths and Difficulties Questionnaire (SDQ) scores, which assesses behavior and emotions, stressful life events, puberty scales, the child-parent relationship, autonomy, sense of belonging to school, and whether the child had a boyfriend/girlfriend. For the suicide attempt model, the top predictors were the SMFQ, SDQ, Spence Anxiety Scale, which assesses the severity of anxiety symptoms, and the CHU9D Index, a measure of health-related quality of life.
Compared to using only a history of self-harm or suicide attempts as predictors, the ML models fared better. Using previous self-harm to predict repeat self-harm achieved an AUC of 0.645, a previous suicide attempt to predict a repeat attempt an AUC of 0.630, and self-harm predicting a suicide attempt an AUC of 0.647.
What surprised the researchers was that previous suicide or self-harm attempts were not a high-risk factor and that environment played such an important role.
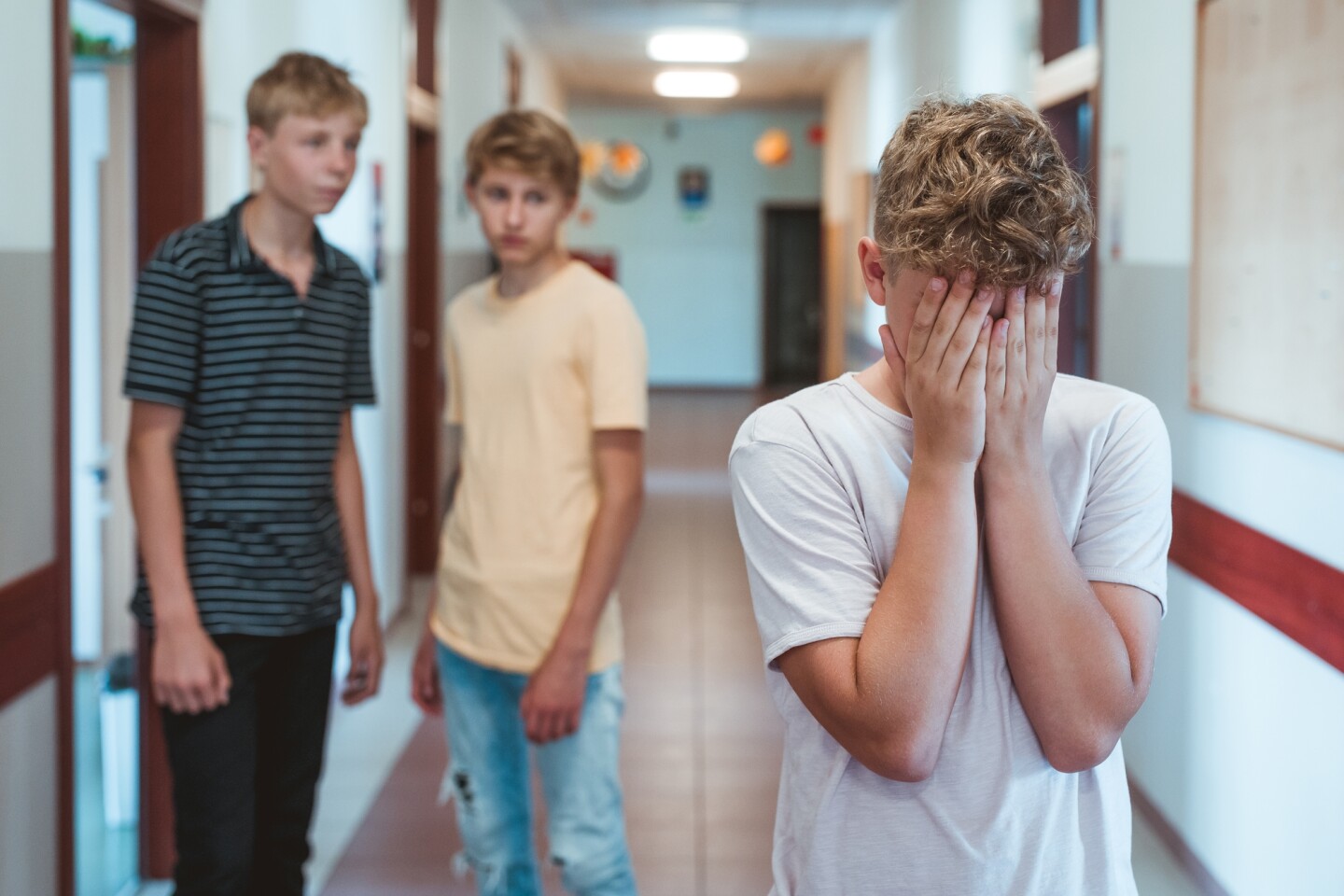
“It was surprising for us to see that previous attempts were not among the top risk factors,” Lin said. “We found that the young person’s environment plays a bigger role than we thought. This is a good thing from the standpoint of prevention, because we now know that there’s more we can do for these individuals.”
The researchers also noted that there were unique factors specific to either suicide or self-harm.
“A unique predictor of suicide was lack of self-efficacy, when someone feels a lack of control over their environment and their future,” said Lin. “And a unique predictor of self-harm was lack of emotional regulation.”
The researchers say their findings are important because they tend to disprove the stereotype that people commit suicide or self-harm solely due to poor mental health. They say their model could be used to assess individualized risk in adolescents.
“Based on patient information, the ML algorithm could calculate a score for each person, and that could be integrated into the electronic medical records system,” Lin said. “The clinician could quickly retrieve that information to confirm or tweak their assessment.”
More research is needed before these models can be rolled out in a clinical setting. They need to be applied to real-life clinical databases to validate their effectiveness at predicting suicide and self-harm attempts.
“As researchers, we will try to continue to generate more information and more evidence,” said Lin. “This is the way to convince stakeholders – clinicians, families, patients and the community – that these data-driven approaches are valuable.”
The study was published in the journal Psychiatry Research.
Source: UNSW Sydney