A collective of human right groups has called for ethical frameworks to be established that can guide the development of machine learning algorithms to ensure discrimination does not become embedded into new artificial intelligence technologies.
Drafted by Amnesty International and Access Now, with subsequent endorsement from the Wikimedia Foundation and Human Rights Watch, The Toronto Declaration seeks to address the risks of human right harms that can result from the use of machine learning systems.
"The Toronto Declaration is unique in setting set out tangible and actionable standards for states and the private sectors to uphold the principles of equality and non-discrimination, under binding human rights laws," says Anna Bacciarelli, from Amnesty International.
The Declaration lays out a comprehensive framework to guide both governments and private companies in the development and implementation of artificial intelligence technologies. The action is an interesting step for human rights organizations as they begin to grapple with the social implications of certain advanced technologies being integrated into social spaces.
Some of the suggestions in the Declaration include private companies taking into account the risk of bias being introduced into a system through incomplete machine learning training data, and governments assessing potential discriminatory outcomes when acquiring and deploying these technologies.
Despite the largely symbolic nature of the Declaration, this is a timely move for human rights groups to make. There have undoubtably been numerous occurrences of problematic bias seeping into technologies steered by machine learning algorithms.
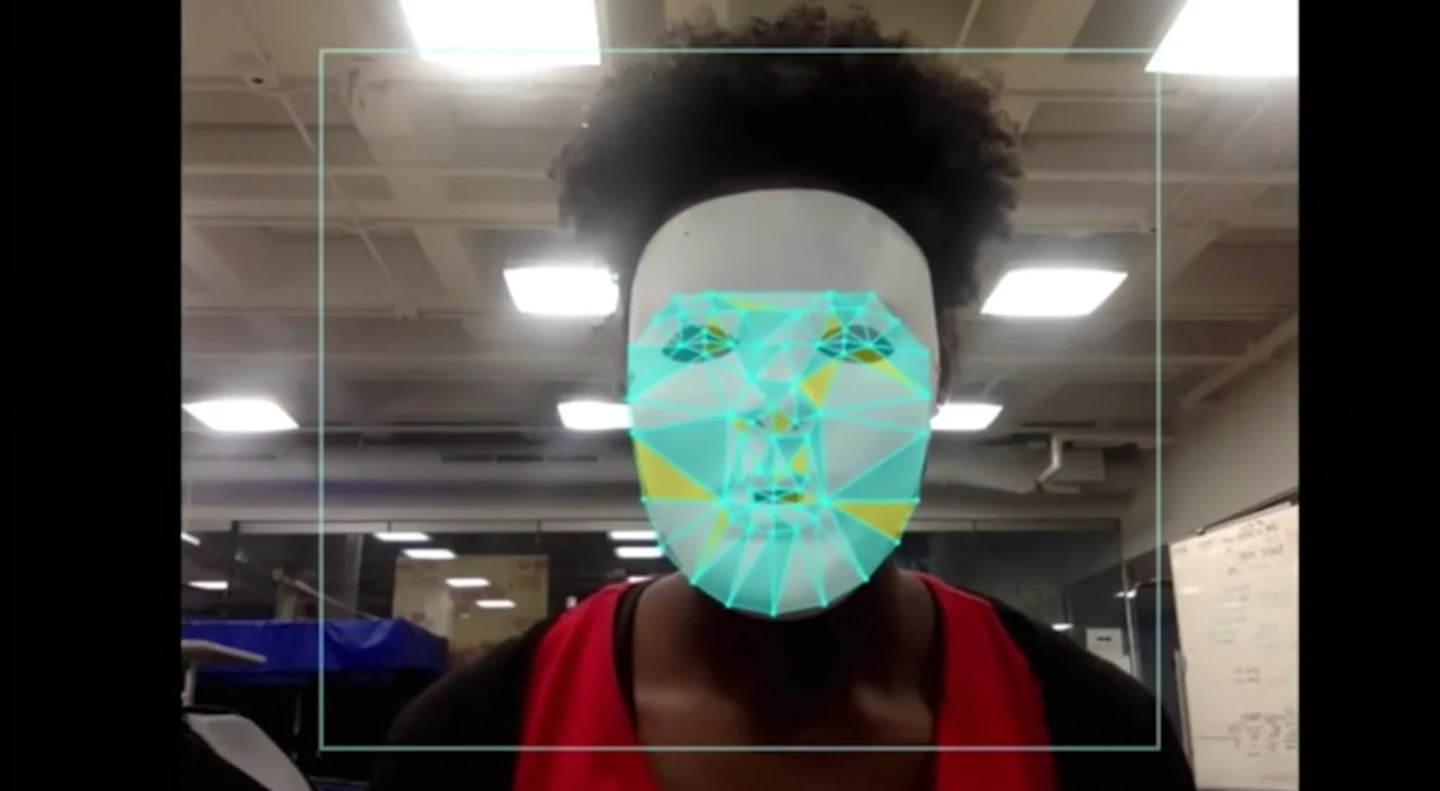
Earlier this year, a study from MIT and Stanford University researchers revealed that three major facial recognition systems returned significant error rates on any subject that wasn't white or male. It was suggested that these flawed systems were generated by machine learning algorithms trained on datasets that contained high volumes of white males over other gender and racial types. The concerns are clear when one realizes these facial recognition systems are already being utilized by law enforcement agencies and health care departments.
Perhaps more worryingly was a report in 2016 revealing that a computer program, used by US courts to inform judges on the risk of defendants reoffending, was dubiously classifying black people as twice as likely to reoffend as white people. The system, called Correctional Offender Management Profiling for Alternative Sanctions (Compas), has been guiding judge's sentences for years, but this report starkly suggested its inbuilt racial bias was perpetuating prejudice in the criminal justice system.
The company behind Compas disagreed with the report and claimed the system bases its risk assessments on a variety of questions, none of which specifically query race. The report, on the other hand, looked at the data, examining the risk scores attributed to 7,000 people, and followed up for several years to compare those scores to the reality of whether they actually reoffended. Despite the algorithms not specifically addressing race, the bias still seemed present, with the system wrongly labeling black defendants as potential reoffenders at double the rate of white defendants.
Although the Toronto Declaration is not currently legally binding, the authors stridently suggest it should be, as it is fundamentally based on international human rights law, which is an established legal framework. This is just a small first step, but a potentially important one, as more and more global systems begin to be controlled by artificial intelligence trained on machine learning algorithms.
Source: Access Now