One of the major anticipated applications for robots is in care for the elderly and helping them with daily tasks. This means that robots have got to adapt to human lifestyles, not the other way around, because granny can’t be expected to program the robot or rearrange her house to suit the machine’s limitations. The Carnegie Mellon University Robotics Institute’s Lifelong Robotic Object Discovery (LROD) project aims to address this by developing ways to use visual and non-visual data to help robots to identify and pick up objects so they can work in a normal human environment without supervision.
One problem with household robots is that robots in general have a lot of trouble dealing with unstructured environments or, as we like to call it, the real world. They prefer places that are open, geometric and with all sorts of nice contrasts so objects are easy to differentiate. This is particularly true if a robot relies solely on vision to identify objects. The traditional approach has been for programmers to build digital models and images of objects that the robot will look for and manipulate, but this isn't easy in the everyday world and this manual approach is time consuming and labor intensive.
The LROD project goes beyond vision by adding location, timestamps, shapes and other factors to help the robot build a realistic map of the world for itself. This “metadata” helps to streamline the process and allows the robot to continually discover and refine its understanding of objects.
The robot used by the Robotics Institute is the Home-Exploring Robot Butler or HERB. This two-armed, mobile robot is equipped with color video and a Kinect depth camera and is able to process non-visual information, such as time and distance traveled. HERB uses a form of LROD, called HerbDisc, which helps it to discover objects on its own and allows it to refine its models of objects on the go so that they’re more relevant to achieving its goals.
Think about how you look for something. It isn't just vision that’s used. You think about where you are, when you last saw the object or one similar, what time it is, how large it is, whether it moves, it’s approximate shape and so on. Figuring out how you’ll pick it up relies on similar cues. This is called “domain knowledge.” HERB uses a similar approach.
The tests were set in a laboratory designed to simulate a cluttered and populated office. What HERB did was to wander around the office looking for possible objects and noting locations where objects might be, such as the floor, tables and cupboards. The first scans basically take in everything where everything seems like an object.
Instead of processing massive amounts of visual data and then matching it against pre-programmed digital models, the robot would go through a series of steps to separate objects from non-objects, figure out what the objects are, and then how to lift them. It did this by scanning the area in red, green and blue and adding three-dimensional data collected from the Kinect sensors.
It then used other “metadata,” such as time and location, to break up any candidates for objects and studied them. Were they on a table where objects are more likely to be? Are they part of the table? What color are they? Are they flat? Do they move? When are they there? And so on. In this way, HERB was able to tell the difference between a frying pan, a jug of milk and a human being, and figure out how to pick them up – though the people were generally left where they were.
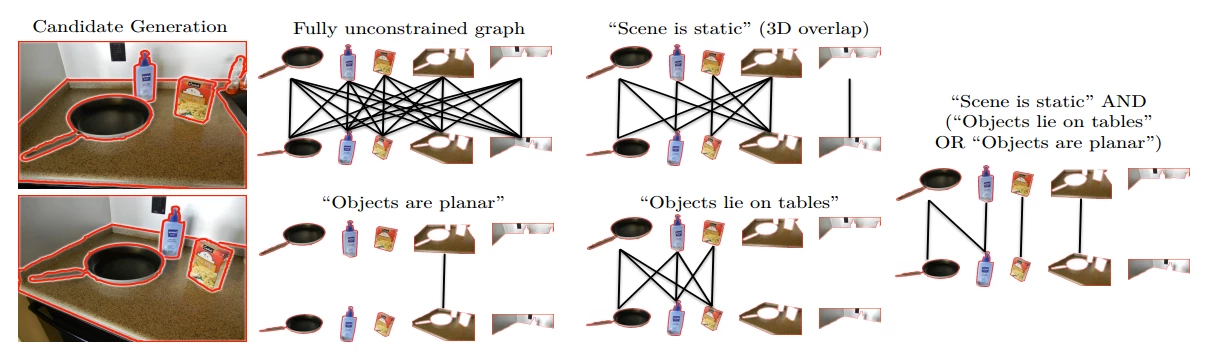
In a way, its a bit like a chess program. It’s possible to beat a human opponent by the brute force method of checking the outcome of every possible move, but it’s more efficient to study various chains of moves and categorize them. HERB does something similar as it splits up object candidates, sorts out the context of where they are and sticking them into categories so it can tell the milk jugs from the people.
This approach allowed HERB to cut the computer processing time by a factor of 190 and triple the number of objects it could identify. It was also able to process hundreds of thousands of samples in under 19 minutes and discover hundreds of new objects over six hours of continuous operation.
Sometimes it even surprised the researchers, such as the time when students left behind a pineapple and a bag of bagels from their lunch and by the next morning HERB had digitally modeled them and sorted out how to pick them up.
“We didn't even know that these objects existed, but HERB did,” said Siddhartha Srinivasa, associate professor of robotics and head of the Personal Robotics Lab, where HERB is being developed. “That was pretty fascinating.”
The future for LROD will see HERB given the ability to identify sheets of paper once it is equipped with better manipulators to allow it to pick them up. After that, internet connections will be provided to allow for crowdsourcing and accessing image libraries to help the robot identify parts of an object that it can’t see or to learn the names of objects.
The team's findings were presented on May 8 at the IEEE International Conference on Robotics and Automation in Karlsruhe, Germany.
The video below shows HERB scanning a room.
Sources: Carnegie Mellon University, Robotics Institute (PDF)