MIT's Improbable Artificial Intelligence Lab has developed a Dexterous Ball Manipulation with a Legged Robot (DribbleBot) that can dribble a soccer ball under real-world conditions similar to those encountered by a human player.
Robot soccer (football to some) has been around since the mid-1990s, though these matches have tended to be a fairly simplified version of the human game. However, getting a robot to manipulate a ball is also a very attractive research topic for roboticists.
Usually, these research efforts have centered on wheeled robots playing on a very flat, uniform surface chasing a ball that it allowed to roll to a halt. For DribbleBot, the team used a quadruped robot with two fisheye lenses and an onboard computer with neural network learning capacity for tracking a size 3 soccer ball over an area that has the uneven terrain of a real pitch and includes sand, mud, and snow. This not only made the ball less predictable as it rolled, but also raised the danger of falling down, which the 40-cm (16-in) tall robot had to recover from and then retrieve the ball like a human player.
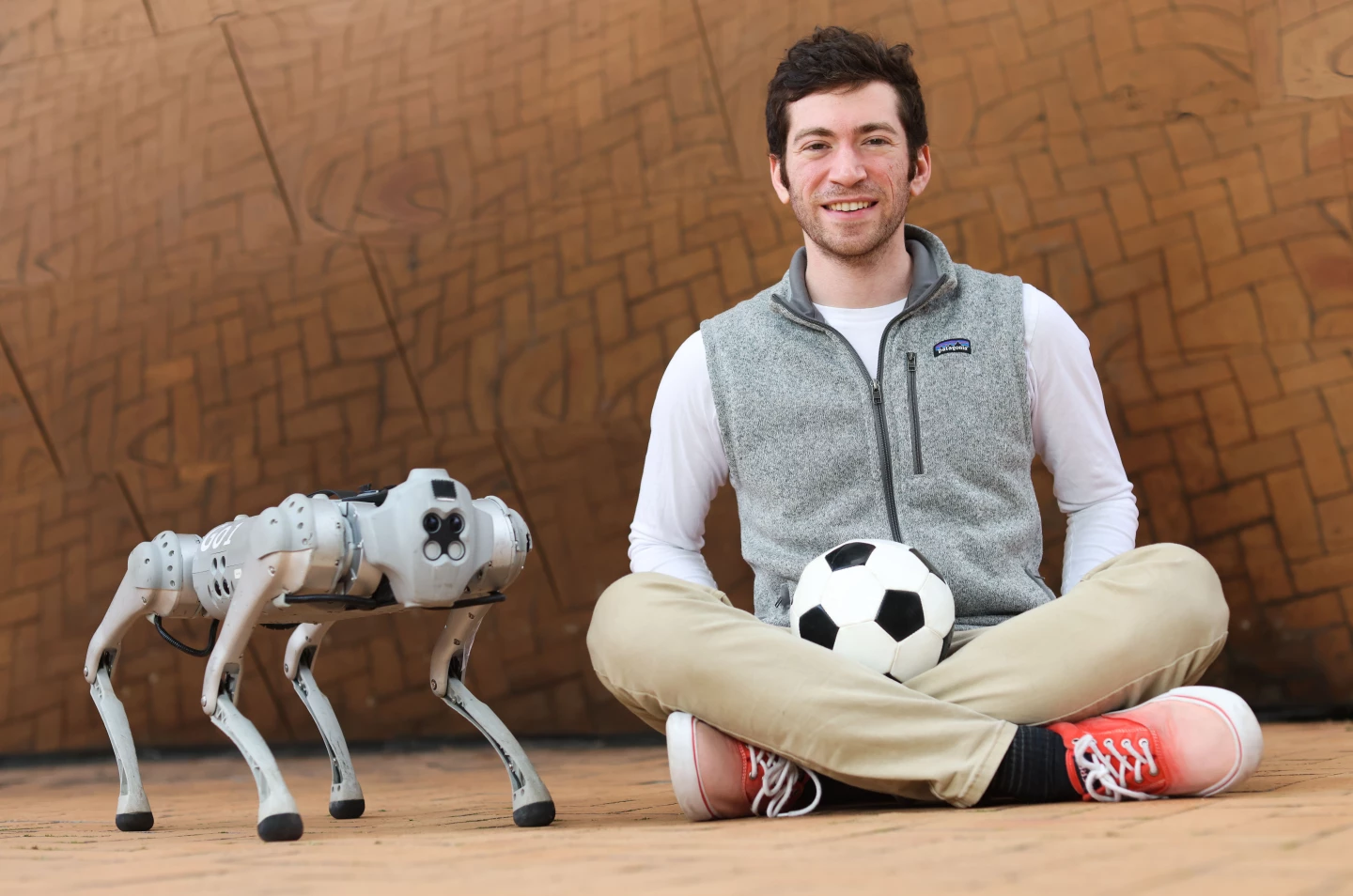
This may seem simple in a world where Boston Dynamics robots are regularly shown running about on broken ground and doing back flips, but there is a big difference in dribbling. A walking robot can rely on external visual sensors and to keep its balance it relies on analyzing how well its feet are gripping the ground. A ball rolling on uneven terrain is much more complex as it responds to small factors that don't affect the dribbler, requiring the robot to discover for itself the skills needed to control the ball while both the ball and it are on the go.
To speed up this process, 4,000 digital simulations of the robot, including the dynamics involved and how to respond to the way the simulated ball rolled, were conducted in parallel in real time. As the robot learned to dribble the ball, it was rewarded with positive reinforcement and received negative reinforcement if it made an error. These simulations allowed hundreds of days of play to be compressed into only a couple.
Then in the real world, the robot's onboard camera, sensors, and actuators allowed it to apply what it had learned digitally and hone those skills against the more complex reality.
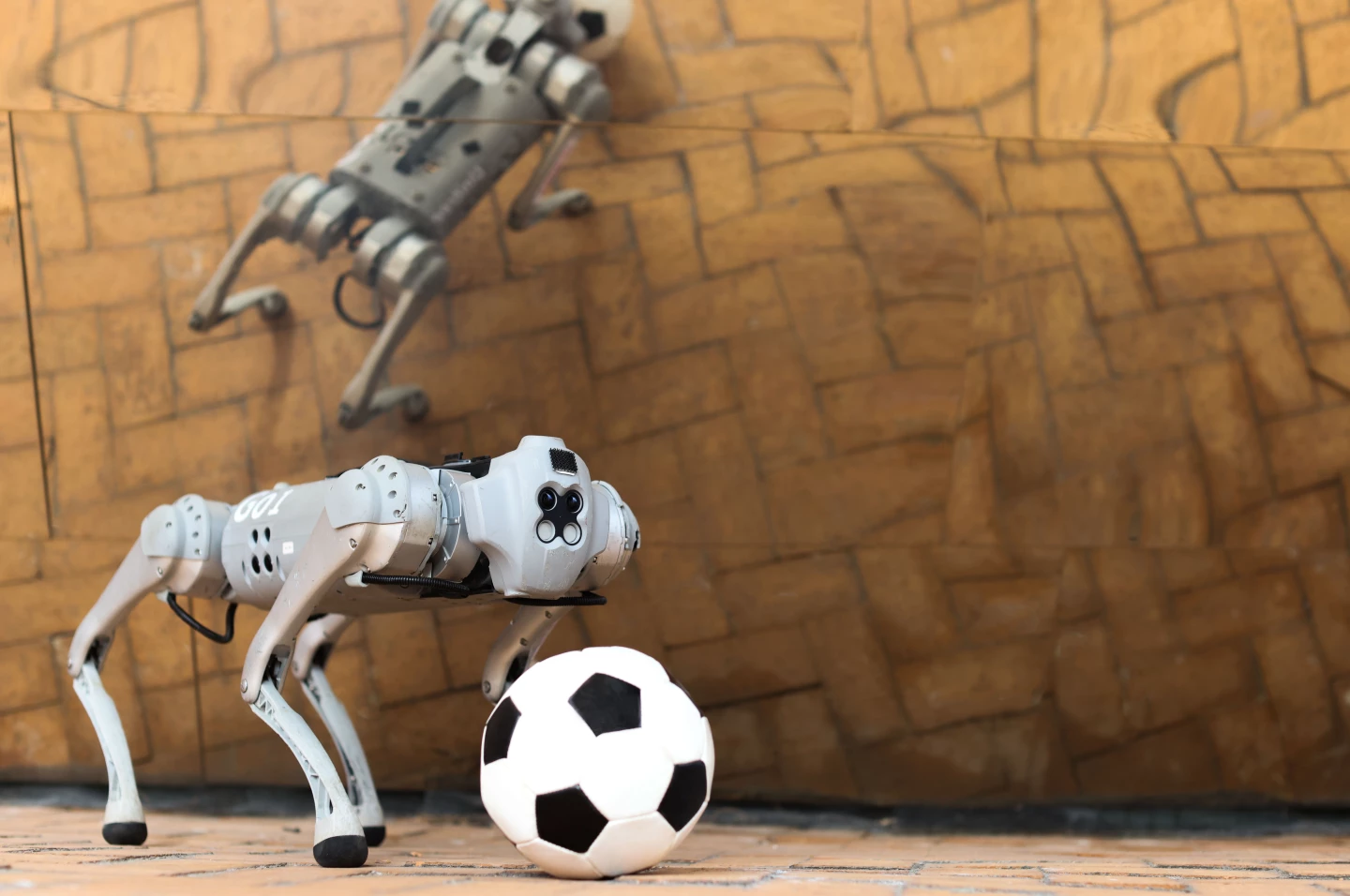
"If you look around today, most robots are wheeled," says Pulkit Agrawal, MIT professor, CSAIL principal investigator, and director of Improbable AI Lab. "But imagine that there's a disaster scenario, flooding, or an earthquake, and we want robots to aid humans in the search-and-rescue process. We need the machines to go over terrains that aren't flat, and wheeled robots can't traverse those landscapes. The whole point of studying legged robots is to go terrains outside the reach of current robotic systems. Our goal in developing algorithms for legged robots is to provide autonomy in challenging and complex terrains that are currently beyond the reach of robotic systems."
The research will be presented at the 2023 IEEE International Conference on Robotics and Automation (ICRA) in London, which begins on May 29, 2023.
The video below discusses DribbleBot.
Source: MIT