If we're ever going to have robot butlers, then they're going to have to learn how to figure things out for themselves. After all, if you have to reprogram the robot for every slight variation on a task, you might as well do it yourself. Scientists at Cornell University's Personal Robotics Laboratory are tackling the formidable challenges posed by "machine learning" by programing robots to observe new situations and proceed accordingly, based on what they already know from the past.
Cornell has already developed a Kinect-based system that can identify peoples' activities based on their movements. If installed in a home care robot, the machine could use it to check that the human in its care was getting enough to drink, brushing their teeth regularly, and so on.
More recently-reported research deals with getting robots to generalize. Instead of only identifying one specific cup as a cup, for instance, the team is trying to get robots to identify a wide range of cups as cups, based on certain features that they all share. It could then go on to identify the handle on any one of those cups, and pick it up using that handle.
Picking things up, however, is less difficult for a robot than putting them down - after all, when placing an object, the robot must ascertain that the surface is stable. It will also place objects in different orientations, depending on the surface. Plates, for instance, lie flat on a table but sit vertically in a dishwasher.
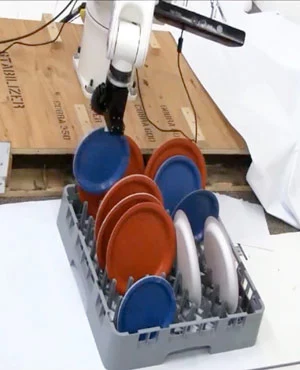
In tests at Cornell, machine learning-programmed robots properly placed a plate, mug, martini glass, bowl, candy cane, disc, spoon and tuning fork on a flat surface, on a hook, in a stemware holder, in a pen holder and on several different dish racks. While the robots had a 98 percent success rate when dealing with objects and environments they had seen before, they still managed to get it right 95 percent of the time when dealing with new objects in new environments.
The researchers are also working on ways of getting robots to take stock of a room when they enter. Part of their work has involved programming a robot with 24 office scenes and 28 home scenes, in which most of the objects were labeled. When entering a room, that robot uses its 3D camera to perform a scan of the entire space, and identifies objects by comparing them to those in its memory. It then breaks that scan up into labeled segments, based on relationships between objects.
The robot knows, for instance, that keyboards are found underneath monitors. This knowledge allowed it to locate a keyboard in a room by first identifying the more easily-spotted monitor, then looking underneath it. By examining an object's color, texture, neighboring objects and features shared with other objects, the robot was able to accurately identify home objects about 83 percent of the time, and office objects about 88 percent.
Needless to say, it becomes apparent what an almost unfathomable amount of work would have to go into a truly human-minded robot like C-3PO or Robby.
The video below shows how the one Cornell robot went about finding the keyboard.