Traditional study of animal behavior is a slow, and imprecise process, with human observers spending months, or even years, watching and classifying different actions. A team from Columbia University is determined to develop an easier, and more objective method using machine learning and algorithms often used for spam-filtering purposes.
Hydra is a genus of primitive freshwater organisms thought to be a close relative of coral, jellies and sea anemones. The transparent body of Hydra allows researchers to observe its entire nervous system, making it a perfect organism for study that connects behavioral actions to nervous system activity.
Earlier work from the Columbia University team successfully recorded Hydra firing neurons in real-time, and connected firing patterns to specific distinct behaviors. This new study set out to comprehensively catalog all the organism's behaviors, and it was in this task that a novel machine learning method was developed.
"People have used machine learning algorithms to partly analyze how a fruit fly flies, and how a worm crawls, but this is the first systematic description of an animal's behavior," says senior author on the study, Rafael Yuste. "Now that we can measure the entirety of Hydra's behavior in real-time, we can see if it can learn, and if so, how its neurons respond."
To study Hydra movements, the researchers used a combination of machine learning and computer vision, applying the classic spam-filtering "bag of words" algorithm to track behavioral patterns. The model analyzes videos of the organism's movements to identify repeating patterns of behavior. Ten behaviors were identified, and the team set out to examine how six of those specific behaviors were affected by the alteration of environmental factors. The results were decidedly unexpected, with the organism barely changing its patterns.
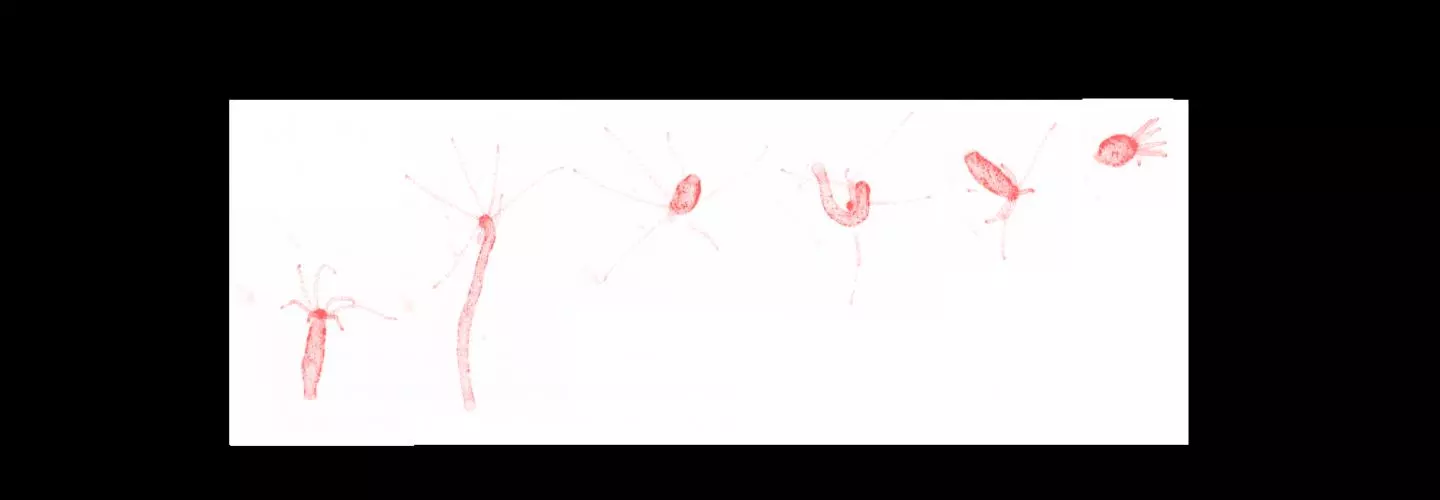
"Whether you fed it or not, turned the light on or off, it did the same thing over and over again like an Energizer bunny," says Yuste.
While these results offer scientists a compelling insight into this specific organism's unexpectedly stable behaviors, it is the novel method used in the study that may be the most exciting outcome. Future research is planned to examine how the organism responds to other stimuli and how its neuronal firing patterns can create stable behavioral responses regardless of external conditions. The pragmatic outcome is that this research could help engineer stable navigation systems for ships or planes that require precise control across variable environmental conditions.
An even greater potential outcome from the research is the possibility that this kind of machine learning behavioral classification system could be applied to more complex animal systems. As the researchers optimistically write in their study, "This would allow scientists to systematically perform experiments to understand how the nervous system controls all animal behavior, a goal that it is the holy grail of neuroscience."
The study was published in the journal eLife.
Source: Columbia University