Researchers at the University of California, San Diego (UCSD) have developed a pedestrian detection system they claim performs in near real-time at higher accuracy than existing systems. The researchers believe that the algorithm and technology could be used in self-driving vehicles, robotics, and in image and video search systems.
The system was developed by electrical engineering professor Nuno Vasconcelos in the UCSD Jacobs School of Engineering. His team combined traditional computer vision models with deep learning to improve accuracy and speed.
The goal was real-time vision that would allow the system to recognize and categorize objects, especially humans, in normal urban driving conditions. This would allow a self-driving car, delivery robot, or low-flying drone to detect and avoid pedestrians and potential conflicts and congestion.
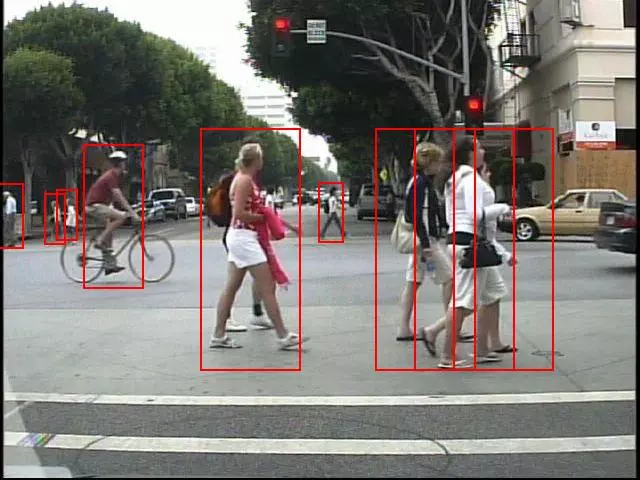
Most pedestrian detection systems divide an image into small sections (referred to as "windows") that are processed by a classification program to determine the presence of a human form. This can be challenging for engineers because humans come in various shapes and sizes and distance changes the perspective and size of objects. In a typical real-time application, this involves processing millions of these windows at 5-30 frames per second.
The cascade detection technique employed in the UCSD system does the same basic function, but does it in stages rather than in one go. This allows the algorithm to quickly discard frames that have no likelihood of containing a human form and concentrate on those that may. So frames that have relatively uniform shapes and colors (the sky, for example) are ignored in favor of frames that are busier.
The second stage classifies and discards frames that have objects similar in shape or color variance to humans, but are not pedestrians (trees, shrubs, other vehicles). The final stages classify in finer and finer detail until just pedestrians are left and marked. Although these final calculations and processes are processor-heavy, only a few of them are required by comparison, so it is done quickly.
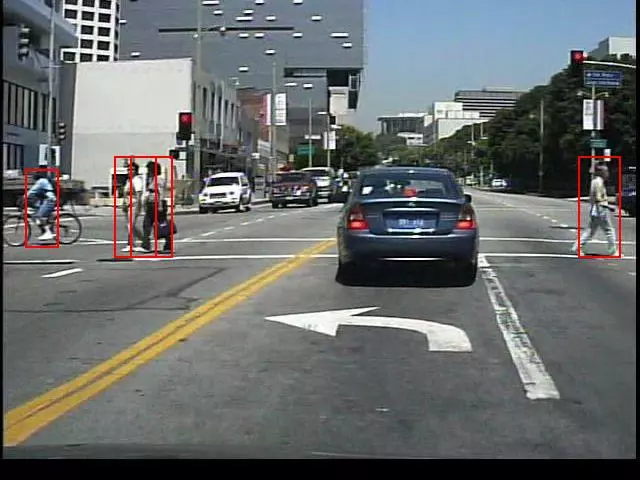
Traditionally, cascade detection systems use simple classifiers, referred to as "weak learners." In the UCSD system, the later-stage detection systems learn as they go, so the classifiers get more and more sophisticated and thus faster. The classifiers in each stage thus get more robust over time and are not all the same from one stage to the next, which is a key difference between this new algorithm and current systems for pedestrian detection.
The algorithm does this, says Vasconcelos, by learning which combinations of weak learners were able to detect the pedestrians in one frame and put more emphasis on those as frames progress, quickening the detection process. The goal is to continually optimize the trade-off between detection accuracy and speed.
For now, the algorithm works only in binary (yes/no) detection tasks, but the UCSD team hopes to extend its capabilities to detect multiple object types simultaneously.