In 2019 we got the first-ever direct image of a black hole, which resembled a fuzzy, orange donut. Now, the team has refined the iconic image with the help of machine learning algorithms to produce the highest resolution image possible with the original data.
Astrophysicists have suspected the existence of black holes for decades, and while there’s mountains of indirect evidence for them, direct images remained elusive. After all, how can you take a photo of something from which light itself cannot escape?
The answer is contrast – the intense gravitational influence of black holes heats up material around them, creating a bright disk that highlights the infinitely dark object in the center. Using this method, the Event Horizon Telescope (EHT) collaboration finally produced the first direct image of a black hole in 2019, capturing the supermassive monster at the center of the galaxy M87.
The image shows a distinct orange ring surrounding a black heart, confirming hypotheses about what black holes would look like. Groundbreaking as it was though, the image was quite blurry, so now the EHT team has sharpened it up.
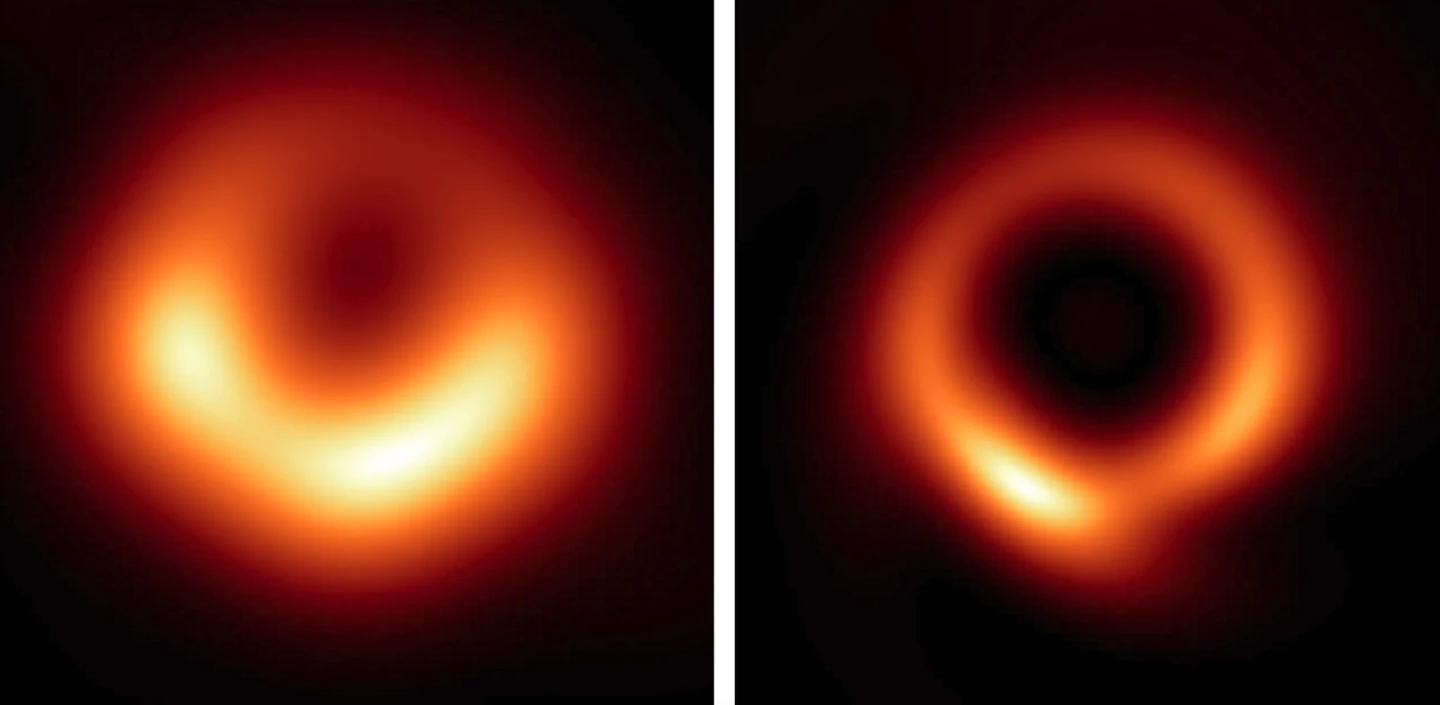
The key to the new and improved image was a machine learning algorithm called principle-component interferometric modeling (PRIMO). The original image was taken by pooling data from radio telescopes around the world to create an Earth-sized virtual telescope, but doing so inevitably has holes in the image since you can’t cover the planet’s surface in telescopes.
PRIMO is designed to patch up those holes. This machine learning system was trained on over 30,000 simulated images of black holes with accretion rings, including a wide range of different models. Recurring patterns were sorted by how often they occurred, and then blended together to form images of what black holes should look like. The original EHT observations were compared to these images, to estimate what the missing structures in the image would look like.
The end result is a much sharper image of M87’s central black hole, stripping away the noise to see a larger, darker black hole and a glowing ring that’s only about half as wide as previously seen. This image, the team says, reaches the maximum resolution you could achieve if you did manage the impossible feat of building an Earth-sized radio telescope.
The new image can help scientists improve models of black hole physics and gravity, and the technique could be applied to other images like the one the EHT collaboration took of the Milky Way’s own supermassive black hole last year.
The research was published in the Astrophysical Journal Letters.
Source: Institute for Advanced Study