Fake news is a problem. This is inarguable. However, exactly how to identify and deal with it is a challenge with no really good solution. Google, Facebook and other tech giants are struggling with exactly how to moderate the spread of both outright misinformation, and extremely biased news reporting. Individual fact-checking and moderating of specific articles is an insanely time-consuming process, and one still frustratingly subject to the bias of individual moderators. So, while Facebook, for example, currently has thousands of human moderators working to keep tabs on the content shooting around its platform, it is clear that some kind of technological resource will play an increasingly significant role in the solution.
A team of researchers from MIT's Computer Science and Artificial Intelligence Lab and the Qatar Computing Research Institute has set out to develop a new machine learning system designed to evaluate not only individual articles, but entire news sources. The system is programmed to classify news sources for general accuracy and political bias.
"If a website has published fake news before, there's a good chance they'll do it again," says Ramy Baly, explaining why the team targeted the veracity of entire sources instead of individual stories. "By automatically scraping data about these sites, the hope is that our system can help figure out which ones are likely to do it in the first place."
The first step in developing the system was feeding it data from a source called Media Bias/Fact Check (MBFC). This independent, non-partisan resource classifies news sources based on political bias and accuracy. On top of this dataset, the system was trained to classify the bias and accuracy of a source based on five features: textual, syntactic and semantic article analysis; its Wikipedia page; Twitter account; URL structure; and web traffic.
Perhaps the most comprehensive of these analytical tools is the system's ability to examine individual articles using 141 different values to classify accuracy and bias. These include linguistic features such as hyperbolic or subjective language, and lexicon classifiers that signify bias or complexity. A source's Wikipedia page is also leveraged for signs related to bias, while the lack of a Wikipedia page could suggest a reduction in credibility.
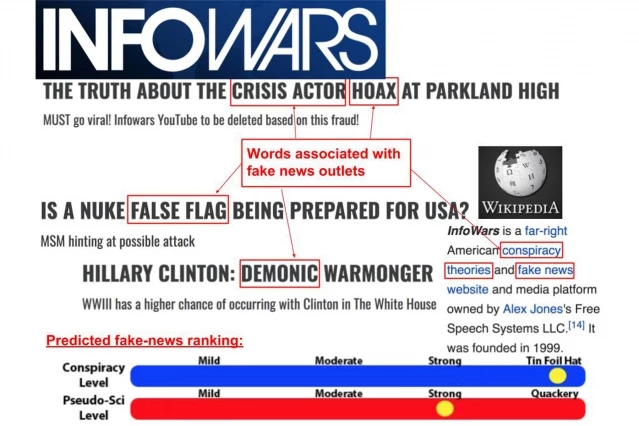
A news source's factual accuracy is ultimately classified using a three point scale (Low, Mixed or High), while political bias is measured on a seven point scale (Extreme-Left, Left, Center-Left, Center, Center-Right, Right, and Extreme Right).
Once the system was up and running it only needed 150 articles from a given source to be able to reliably detect accuracy and bias. At this stage the researchers report it is 65 percent accurate in detecting factual accuracy and 70 percent accurate at detecting political bias, compared to similar human-checked data from MBFC.
It's suggested that despite potential improvements in predictive accuracy that are sure to be developed by improving the algorithm, it is essentially designed to work in tandem with human fact-checking sources.
"If outlets report differently on a particular topic, a site like Politifact could instantly look at our fake news scores for those outlets to determine how much validity to give to different perspectives," explains Preslav Nakov, co-author on the new research.
One outcome the researchers hope to generate from this system is a new app that offers users accurate news stories spanning a broad political spectrum. In being able to effectively separate factual accuracy from political bias, the app could hypothetically present an individual user with articles on a given story that may be from a different political angle than they are commonly exposed to.
"It's interesting to think about new ways to present the news to people," says Nakov. "Tools like this could help people give a bit more thought to issues and explore other perspectives that they might not have otherwise considered."
Absolute trust in the algorithm is not really the ultimate goal here. After all, as the recent bias in facial recognition controversy illustrated, an algorithm will essentially reflect the bias of the humans that create it. So, a machine learning system such as this one is by no means a magic bullet to the problem of fake or biased news, but it certainly presents us with a valuable tool to help manage the ongoing issue.
The new study is available on the preprint journal website Arxiv.
Source: MIT