For decades one of the holy grails of medical diagnostic devices has been the non-invasive glucose monitor. From wrist-watches to contact lenses, the alluring dream of a device to continually monitor blood glucose levels without breaking the skin has failed to become reality, despite a wide variety of promising innovations. Researchers from the University of Warwick are now demonstrating the latest prospective technique using artificial intelligence to detect hypoglycemic events from simple ECG signals.
"[Fingerpricks] are never pleasant and in some circumstances are particularly cumbersome,” explains Leandro Pecchia, corresponding author on the new study. “Taking [fingerprick] during the night certainly is unpleasant, especially for patients in pediatric age. Our innovation consisted in using artificial intelligence for [automatically] detecting hypoglycemia via few ECG beats. This is relevant because ECG can be detected in any circumstance, including sleeping."
The key breakthrough made by the University of Warwick team was to develop an AI system that can learn the ECG rhythms of an individual patient. Prior study investigating blood-glucose tracking through ECG data has been unsuccessful due to the extraordinary variety of signals found in different subjects. Because of the heterogeneous nature of ECG data, no machine learning system has been able to successfully take a large cohort of ECG recordings and find universal patterns to correlate with blood glucose measurements in individuals.
As seen in the image below, the ECG measurements signaling hypoglycemia between two subjects can be incredibly disparate, meaning the only way forward was to develop an AI system that can detect personalized fluctuations in each patient.
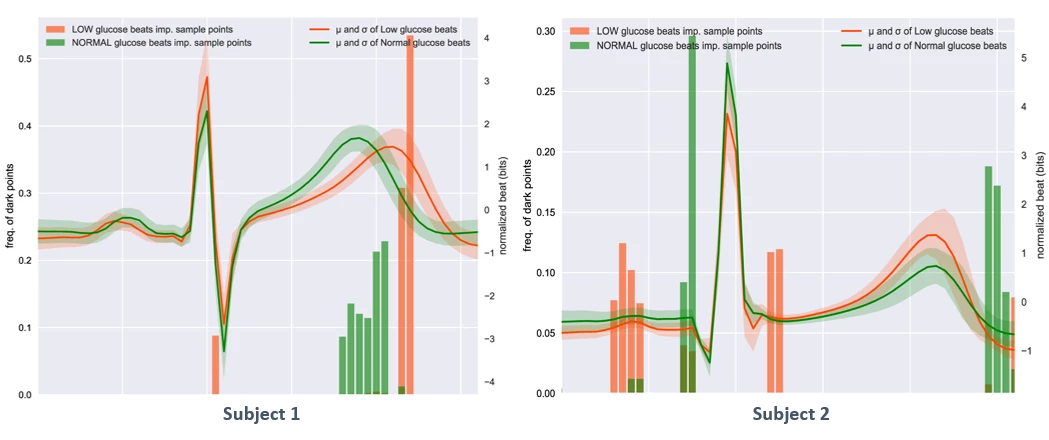
“The differences highlighted above could explain why previous studies using ECG to detect hypoglycemic events failed,” says Pecchia. “The performance of AI algorithms trained over cohort ECG-data would be hindered by these inter-subject differences. Our approach enable personalized tuning of detection algorithms and emphasize how hypoglycemic events affect ECG in individuals.”
In a newly published journal article the researchers report the results of two pilot studies into the efficacy of the novel system. In healthy volunteers the system was able to detect low-glucose events with an accuracy of 82 percent.
Of course, as previously mentioned, this is not the first non-invasive glucose monitoring system to be presented as effective in an early pilot stage. The researchers are pragmatic about how far from the market this system could be, with a great deal more work necessary to verify and refine the technique in larger patient populations. However, the study does note that this ECG framework could be incorporated into a broader blood glucose tracking system that uses other non-invasive physiological signals, such as physical activity levels, skin conductivity and nutrition information.
The new study was published in the journal Scientific Reports.
Source: University of Warwick